- CSCD核心中文核心科技核心
- RCCSE(A+)公路运输高质量期刊T1
- Ei CompendexScopusWJCI
- EBSCOPж(AJ)JST
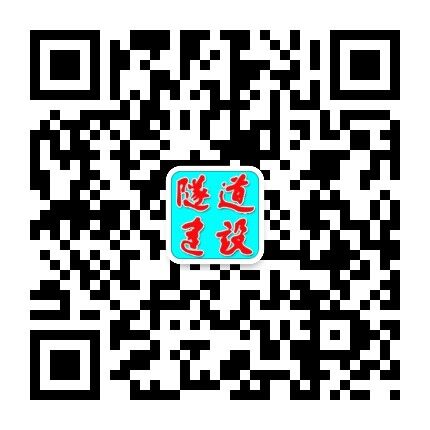
隧道建设(中英文) ›› 2024, Vol. 44 ›› Issue (10): 2032-2040.DOI: 10.3973/j.issn.2096-4498.2024.10.011
关振长1, 谢立夫1, 周宇轩1, 罗嵩2, 许超3
GUAN Zhenchang1, XIE Lifu1, ZHOU Yuxuan1, LUO Song2, XU Chao3
摘要: 为避免盾构轴线偏离引发衬砌管片错台、开裂等质量与安全问题,提出一种基于机器学习算法的盾构姿态智能预测模型与控制方法。以盾构掘进施工的实测数据为驱动,通过贝叶斯优化(BO)与支持向量回归(SVR)构建盾构姿态预测模型,挖掘施工参数-地层信息-盾构姿态三者间的非线性关系。结合模拟退火算法(SA)形成可控施工参数动态调整的盾构姿态控制方法,并将其应用于福州滨海快线南—三区间隧道的工程实践。主要结论如下: 1)经数据预处理、特征筛选及BO超参数优化,基于SVR的盾构姿态预测模型具备优异的预测性能和泛化能力; 2)结合SA算法进行可控施工参数调整时,需设置合理的优化规则,以确保所推荐的可控施工参数具备可操作性; 3)将姿态控制方法应用于南—三区间后续掘进施工以辅助纠偏,盾尾垂直偏差在10环掘进过程中由45 mm减至18 mm,实现了连续稳定纠偏。