- CSCD核心中文核心科技核心
- RCCSE(A+)公路运输高质量期刊T1
- Ei CompendexScopusWJCI
- EBSCOPж(AJ)JST
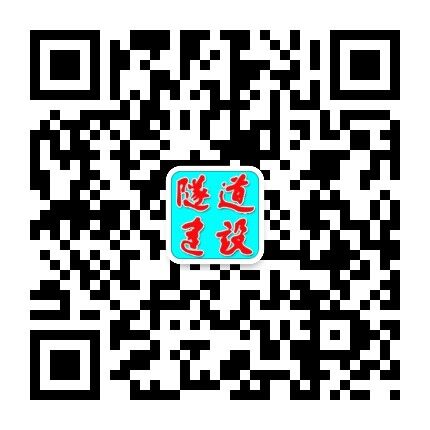
隧道建设(中英文) ›› 2024, Vol. 44 ›› Issue (11): 2171-2180.DOI: 10.3973/j.issn.2096-4498.2024.11.007
雒伟勃1, 李龙1, 汪来2, *, 孙佳利1, 潘秋景2
LUO Weibo1, LI Long1, WANG Lai2, *, SUN Jiali1, PAN Qiujing2
(1. CCCC Third Harbor Engineering Co., Ltd., Shanghai 200032, China; 2. School of Civil Engineering, Central South University, Changsha 410075, Hunan, China)
摘要: 土舱压力是保证盾构隧道施工安全和控制施工风险的关键参数之一。为此,提出一种基于残差神经网络的盾构土舱压力预测方法。首先,通过对南京地铁某盾构区间的掘进参数数据进行收集和分析,构建具有多个残差块的残差神经网络模型。然后,利用所建立的残差神经网络模型对盾构土舱压力进行预测,并评估模型对土舱压力的预测性能。最后,对残差神经网络的关键模型参数(包括残差块数目、网络宽度和学习率)进行参数分析,比较参数变化时土舱压力的预测性能,确定最佳的模型结构。并对模型关键参数进行分析。研究结果表明: 1)所提出的残差神经网络模型可以较准确地预测盾构土舱压力,不同位置的土舱压力预测值与实际值接近; 2)1#、2#、3#、4#、5#和6#土舱压力的决定系数(R2)分别为0.95、0.96、0.94、0.90、0.91和0.96,均方根误差(ERMSE)介于0.017~0.023 MPa; 3)相比于人工神经网络(ANN)、支持向量回归(SVR)和随机森林(RF)模型,残差神经网络模型对土舱压力的预测准确性更高。