- CSCD核心中文核心科技核心
- RCCSE(A+)公路运输高质量期刊T1
- Ei CompendexScopusWJCI
- EBSCOPж(AJ)JST
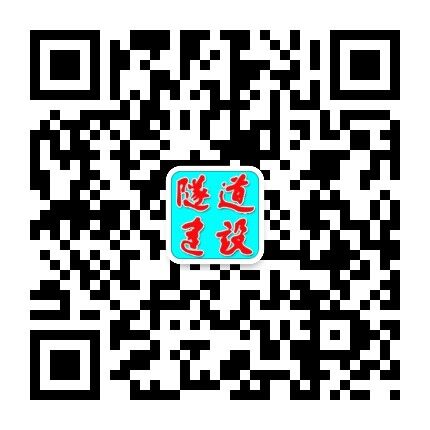
隧道建设(中英文) ›› 2023, Vol. 43 ›› Issue (9): 1549-1557.DOI: 10.3973/j.issn.2096-4498.2023.09.012
程勇1, 王琛2, 刘夏临1, 3, 刘继国1, 3, 陈世纪4, 黄胜4
(1. 中交第二公路勘察设计研究院有限公司, 湖北 武汉 430056; 2. 中国交建总承包经营分公司, 北京 100088; 3. 中国交建隧道与地下空间工程技术研发中心, 湖北 武汉 430056; 4. 中山大学土木工程学院, 广东 珠海 519082)
Application of Machine LearningBased Lithology Identification Analysis for Tunnel Geological Survey
CHENG Yong1, WANG
Chen2, LIU Xialin1, 3, LIU Jiguo1, 3,
CHEN Shiji4, HUANG Sheng4
(1. CCCC Second Highway Consultants Co., Ltd., Wuhan 430056, Hubei, China; 2. China Communications Construction General Contracting and Operation Branch, Beijing 100088, China; 3. CCCC Research and Development Center on Tunnel and Underground Space Technology, Wuhan 430056, Hubei, China; 4. School of Civil Engineering, Sun YatSen University, Zhuhai 519082, Guangdong, China)
摘要: 为提高水平定向钻勘察中岩性识别的效率,基于机器学习算法采用钻进参数识别围岩岩性。以新疆某隧道工程为例,通过对水平定向钻的工作原理进行分析,采用钻进速度、校正后的钻孔底部压强、泥浆压力和进浆流量作为输入特征预测围岩岩性。对KNN(k-nearest neighbor)算法和随机森林算法各设置48个超参数,测试集的平均准确率分别为83.28%和93.04%,模型不存在欠拟合和过拟合问题。将五分类问题转化为5个二分类问题,2种算法的准确率、精确率、召回率、F1值基本均在90.00%以上,受试者工作特征(receiver operating characteristic,ROC)曲线中曲线下面积(area under curve,AUC)也接近于1。〖JP2〗使用Smote过采样后的KNN算法和随机森林算法都具有良好的鲁棒性和泛化能力,但综合各项评价指标可知,使用随机森林模型预测围岩岩性的效果更佳。