- CSCD核心中文核心科技核心
- RCCSE(A+)公路运输高质量期刊T1
- Ei CompendexScopusWJCI
- EBSCOPж(AJ)JST
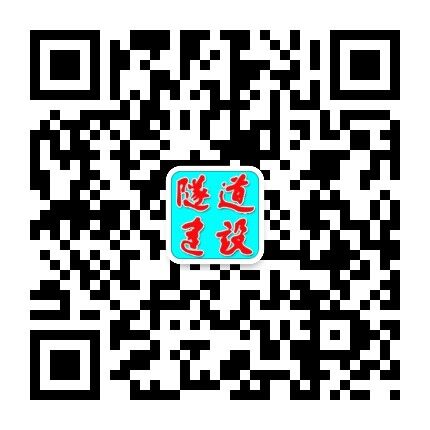
隧道建设(中英文) ›› 2023, Vol. 43 ›› Issue (S1): 222-232.DOI: 10.3973/j.issn.2096-4498.2023.S1.026
刘汭琳1, 满轲1, *, 刘晓丽2, 宋志飞1, 周然3
(1. 北方工业大学土木工程学院, 北京 100043; 2. 清华大学 水沙科学与水利水电工程国家重点试验室, 北京 100084; 3. 粤水电轨道交通建设有限公司, 广东 广州 510610)
Prediction of Ground Conditioning Effect of WaterRich Sandy Stratum Based on Genetic AlgorithmBack Propagation Neural Network
LIU Ruilin1, MAN Ke1, *, LIU Xiaoli2, SONG Zhifei1, ZHOU Ran3
(1. College of Engineering, North China University of Technology, Beijing 100043, China;2. State Key Laboratory of Hydroscience and Hydraulic Engineering, Tsinghua University, Beijing 100084, China;3. YSD Rail Transit Construction Co., Ltd., Guangzhou 510610, Guangdong, China)
摘要: 为了解决通过室内试验评价渣土改良效果存在耗时长、成本高,无法满足目前隧道掘进中的预测需求等问题,基于深度学习,引入遗传算法GA(genetic algorithm)对传统BP(back propagation)神经网络重新设计和优化,创建GA-BP模型;选择均方根误差GMSE、平均绝对误差MAE和决定系数R2作为评价模型预测效果的研究指标; 利用机器学习中支持向量机算法与随机森林算法的预测结果与GA-BP模型的预测结果进行对比。试验结果表明: 1)基于深度学习的传统BP模型和本文创建的GA-BP模型的各项评价指标皆高于机器学习算法; 2)相较于传统BP网络的预测结果,GA-BP模型对内摩擦角、渗透系数和坍落度预测的最高相对误差分别降低了7.18%、5.02%、1.17%,且GA-BP模型的RMSE、MAE和R2值都优于传统BP模型和机器学习算法的预测结果。由此可得,基于深度学习的神经网络比机器学习算法更能提取到数据之间的关联性,且经过遗传算法优化后得到的GA-BP模型提高了传统BP模型的预测准确度。