- CSCD核心中文核心科技核心
- RCCSE(A+)公路运输高质量期刊T1
- Ei CompendexScopusWJCI
- EBSCOPж(AJ)JST
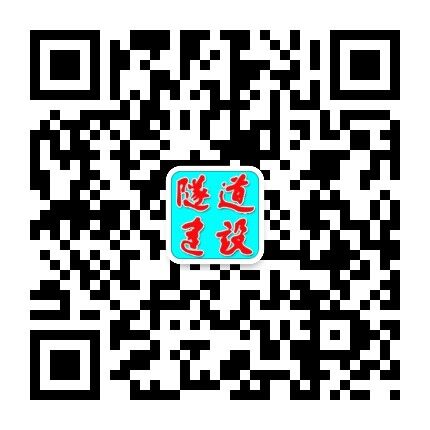
隧道建设(中英文) ›› 2024, Vol. 44 ›› Issue (6): 1274-1282.DOI: 10.3973/j.issn.2096-4498.2024.06.014
周梦琳1, 陈强1, *, 汪波2, 宋自愿1, 彭传阳1, 程黎1
ZHOU Menglin1, CHEN Qiang1, *, WANG Bo2, SONG Ziyuan1, PENG Chuanyang1, CHENG Li1
摘要: 针对勘察设计阶段判别的隧道围岩等级与施工过程中实际揭露的围岩情况不符的问题,提出一种以隧道施工期实际揭露的围岩为研究对象的智能动态分级方法。首先,依托甘肃省渭武高速公路木寨岭隧道工程,利用单反相机采集掌子面图像,基于深度学习ResNet18网络设计T-ResNet模型,进行掌子面围岩图像特征定性识别分类; 然后,利用数字图像处理技术定量识别、提取节理裂隙特征参数,进而确定掌子面完整性指标; 最后,结合岩石坚硬程度、岩体完整程度、主结构面产状、地下水发育状况、初始地应力、节理延展性6个指标建立围岩分级指标体系,并采用特征加权KNN(K-nearest neighbor)算法模型实现隧道围岩智能动态分级。研究结果表明: 1)T-ResNet模型在节理裂隙测试集的准确率达到83.23%,在地下水测试集的准确率达到92.86%,可以实现围岩特征的有效识别与精确分类; 2)使用机器视觉方法处理现场围岩图像,可快速提取岩体完整性系数和地下水发育情况,实现现场智能化高效分析; 3)KNN智能动态分级方法在依托工程具有良好的适用性和较高的准确性,可有效实现隧道施工过程中的围岩动态分级。