- CSCD核心中文核心科技核心
- RCCSE(A+)公路运输高质量期刊T1
- Ei CompendexScopusWJCI
- EBSCOPж(AJ)JST
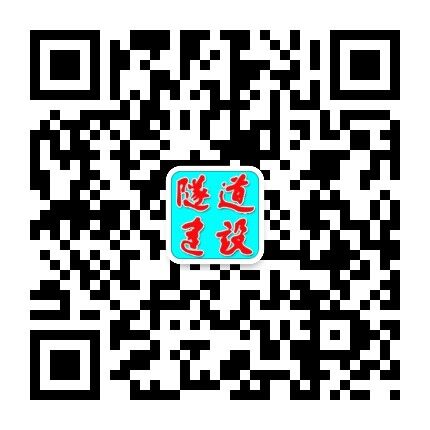
隧道建设(中英文) ›› 2024, Vol. 44 ›› Issue (11): 2223-2232.DOI: 10.3973/j.issn.2096-4498.2024.11.012
郁万浩1, 刘陕南1, *, 肖晓春2
Prediction of Shield Tunneling-Induced Surface Settlement Using Time Series Generative Adversrial Networks Enhanced Convolutional Neural Networks-Long Short-Term Memory Model
YU Wanhao1, LIU Shannan1, *, XIAO Xiaochun2
摘要: 为更准确地预测小数据量下盾构法施工造成的地表沉降,提出基于TimeGAN(time series generative adversarial networks,时间序列生成对抗网络)增强的CNN(convolutional neural networks,卷积神经网络)-LSTM(long short-term memory,长短期记忆网络)盾构掘进地表沉降预测模型,并依托上海北横通道新建工程Ⅱ标盾构施工项目验证该增强模型的性能。首先,选取300环的部分施工参数、地质参数、几何参数以及地表最大沉降,对比LSTM、CNN-LSTM与TimeGAN-CNN-LSTM的性能,证明CNN-LSTM对于盾构施工环境下多参数的预测效果明显优于LSTM,TimeGAN-CNN-LSTM增强模型优于CNN-LSTM; 然后,通过更改训练集及测试集的大小,对不同数据集下TimeGAN-CNN-LSTM增强模型相较CNN-LSTM的预测效果进行研究。结果表明: TimeGAN-CNN-LSTM增强模型预测效果相较CNN-LSTM模型提升显著,且当训练集与测试集比值为4~8时,提升最为显著。