- CSCD核心中文核心科技核心
- RCCSE(A+)公路运输高质量期刊T1
- Ei CompendexScopusWJCI
- EBSCOPж(AJ)JST
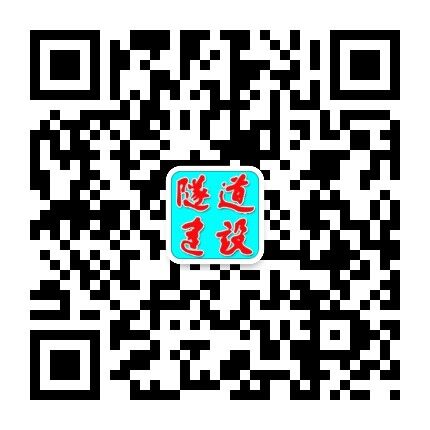
隧道建设(中英文) ›› 2024, Vol. 44 ›› Issue (5): 952-963.DOI: 10.3973/j.issn.2096-4498.2024.05.004
裴成元1, 张云旆2, *, 刘军生1, 刘立鹏2, 曹瑞琅2
PEI Chengyuan1, ZHANG Yunpei2, *, LIU Junsheng1, LIU Lipeng2, CAO Ruilang2
摘要: 针对TBM掘进过程中缺乏对围岩质量和塌方风险快捷、精准的预测预警方法,通过对TBM掘进大数据的深入挖掘,结合对实际工程塌方数据的剖析,提出潜在塌方风险的辅助判断依据。首先,对冗杂、连续的原始采集数据进行预处理,获取高质量的分析数据; 然后,基于参数的相关性分析提出围岩特征参数的计算方法,并围绕特征参数的合理性和适用性,通过理论推导、室内试验和现场原位掘进试验进行论证; 最后,结合实际的TBM塌方案例分析特征参数与围岩地质情况的相关性,提出塌方风险快速判断依据。结果表明: 基于TBM掘进数据获取的围岩特征参数在一定程度上反映了围岩质量,其数值与围岩质量正相关,当其数值显著降低、变幅超过69.2%时,当前的掘进循环极大可能存在塌方风险。