- CSCD核心中文核心科技核心
- RCCSE(A+)公路运输高质量期刊T1
- Ei CompendexScopusWJCI
- EBSCOPж(AJ)JST
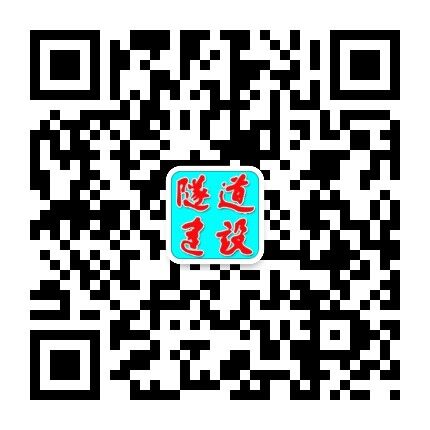
隧道建设(中英文) ›› 2024, Vol. 44 ›› Issue (S1): 478-484.DOI: 10.3973/j.issn.2096-4498.2024.S1.051
袁月明1, 刘洪亮2, 闫宗伟3, 张梓琦2, 郭佩凡3, 张子睿2, *, 杨光3
(1. 山东高速建设管理集团有限公司, 山东 济南 250000; 2. 山东大学, 山东 济南 250100;3. 山东高速临滕公路有限公司, 山东 临沂 273400)
Three-Dimensional Laser Tunnel Crack Detection Based on Federated Weighted Learning Algorithm
YUAN Yueming1, LIU Hongliang2, YAN Zongwei3, ZHANG Ziqi2, GUO Peifan3,ZHANG Zirui2,
*, YANG Guang3
(1. Shandong Hi-Speed Construction Management Group Co., Ltd., Jinan 250000, Shandong, China; 2. Shandong University, Jinan 250100, Shandong, China; 3. Shandong Expressway Linteng Highway Co., Linyi 273400, Shandong, China)
摘要: 为解决采用三维激光扫描仪探测隧道裂缝的识别精度低、抗干扰能力差的问题,提出基于联邦加权学习算法的裂缝探测研究新思路。基于隧道激光点云数据,首先使用优化联邦加权学习算法,并采用异步和残差测试自适应调整算法,以此整体达到精准探测隧道裂缝的目的。在临滕高速隧道进行试验,围绕裂缝探测的可靠性、准确度和测量精度等指标,将本文算法与传统算法进行对比分析,结果表明本文提出的新方法能有效提高隧道裂缝探测的可靠性及准确率,对探测裂缝宽度的精度上也有较好的性能。当探测结果中出现灰尘、钢筋裸露等干扰因素时,新算法较传统算法在可靠性上仍有明显优势,仍能达到95%以上的识别准确度和低于10%的识别误识率,这些确保了算法应用效果的鲁棒性。通过工程现场的实践,本算法识别出的裂缝宽度与人工测量值之间最小偏差仅为0.06 mm,验证了其良好的裂缝识别精度。