- CSCD核心中文核心科技核心
- RCCSE(A+)公路运输高质量期刊T1
- Ei CompendexScopusWJCI
- EBSCOPж(AJ)JST
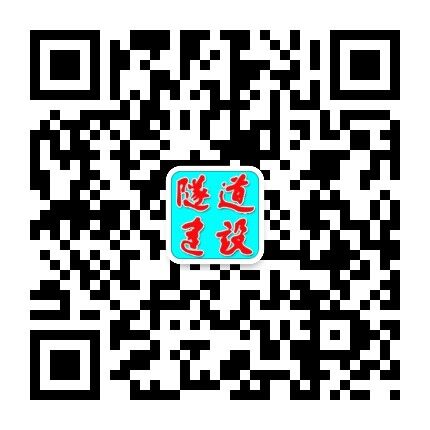
隧道建设(中英文) ›› 2024, Vol. 44 ›› Issue (7): 1520-1531.DOI: 10.3973/j.issn.2096-4498.2024.07.018
武斌, 于双玲, 陈杨杨, 赵洁*
WU Bin, YU Shuangling, CHEN Yangyang, ZHAO Jie*
摘要: 为进一步研究现有公路隧道裂缝检测算法中对裂缝特征提取不充分、抗干扰能力弱而导致漏检以及检测速度慢等问题,提出一种基于改进YOLOv5的隧道裂缝检测方法。首先,在主干网络中结合高效的三维无参数注意力机制,引入残差模块C3SM,用于增强深浅层特征信息之间的交互,在优化计算复杂度的同时增强网络特征提取能力; 其次,在特征金字塔中采用一种新的特征融合网络结构,整合相邻层的特征图,能较好地保留裂缝边缘信息,在保证语义信息不受损失的同时加快模型的检测速度; 最后,采用位置损失函数WIoU优化遮挡和重叠目标的检测效果。为验证该方法的有效性,在Tunnel-crack和湖州隧道裂缝数据集上进行大量试验,结果表明: 所提出检测方法的精度与速度分别达到88.7%、103.5帧/s和85.1%、99.4帧/s,相比大多数高性能目标检测器具有更高的识别准确率,并能满足隧道裂缝检测的要求。