- CSCD核心中文核心科技核心
- RCCSE(A+)公路运输高质量期刊T1
- Ei CompendexScopusWJCI
- EBSCOPж(AJ)JST
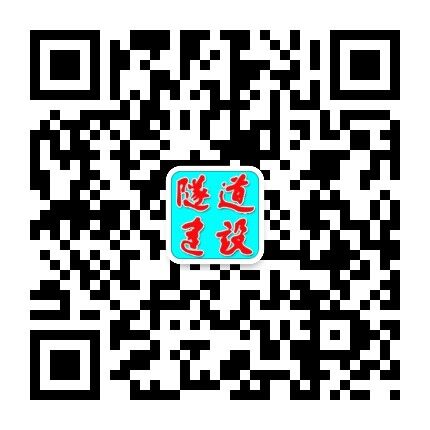
隧道建设(中英文) ›› 2025, Vol. 45 ›› Issue (1): 139-150.DOI: 10.3973/j.issn.2096-4498.2025.01.011
刘哲1, 2, 3, 许超1, 2, 3, 熊栋栋1, 2, 3
(1. 中交第二航务工程局有限公司, 湖北 武汉 430040;2. 交通运输行业交通基础设施智能制造技术研发中心, 湖北 武汉 430040; 3. 长大桥梁建设施工技术交通行业重点实验室, 湖北 武汉 430040)
LIU Zhe1, 2, 3, XU Chao1, 2, 3, XIONG Dongdong1, 2, 3
(1. CCCC Second Harbor Engineering Company Ltd., Wuhan 430040, Hubei, China; 2. Research and Development Center of Transport Industry of Intelligent Manufacturing Technologies for Transport Infrastructure, Wuhan 430040, Hubei, China; 3. Key Laboratory of Large-Span Bridge Construction Technology, Wuhan 430040, Hubei, China)
摘要: 针对盾构姿态预测模型存在易过拟合、预测精度低的问题,提出一种基于融合注意力机制的盾构姿态组合预测模型。为强化有效特征的提取,抑制冗余特征信息的表达,引入基于选择性卷积核网络(selective kernel networks,SKNet)的特征注意力机制提取网络,消除固定尺寸卷积核带来的限制,并自适应形成带有注意力的特征映射。为更好地捕捉长期信息和特征模式,通过双向长短期记忆网络(bidirectional long short-term memory,BiLSTM)、门控循环单元(gated recurrent unit, GRU)得到2组隐含输出结果,再利用多头注意力机制,捕获组合模型输出的隐含特征与模型输出的盾构姿态之间的依赖关系,进一步提高预测模型对重要隐含特征的信息抓捕能力; 同时,为解决地质勘察钻孔数据连续性差、精确性不足,难以应用于机器学习模型训练的问题,将基于人工先验知识的二级特征引入模型特征输入,提升模型对地层信息的感知能力。最后,基于广州地铁12号线官洲站—大学城北站盾构实例,对模型不同参数结构下的性能进行研究,并进行对比试验验证模型性能,采用可解释性试验评估特征对预测结果的影响。试验结果表明,相比其他预测模型,所提出的预测模型优越性更好,预测精度更高,解决了长时间序列高特征维度数据在传统模型下易过拟合且预测精度较低的问题。