- CSCD核心中文核心科技核心
- RCCSE(A+)公路运输高质量期刊T1
- Ei CompendexScopusWJCI
- EBSCOPж(AJ)JST
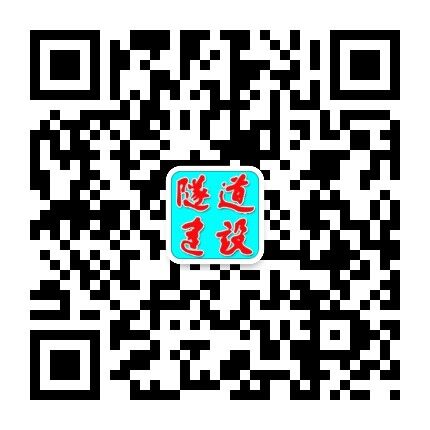
隧道建设(中英文) ›› 2022, Vol. 42 ›› Issue (8): 1443-1452.DOI: 10.3973/j.issn.2096-4498.2022.08.014
梁铭, 彭浩, 解威威*, 宋冠先, 朱孟龙, 张亚飞
DoubleLayer Quality Evaluation of Surrounding Rock of a Tunnel Based
on Advance Drilling and Optimized Integration Algorithm
LIANG Ming, PENG Hao, XIE Weiwei*,
SONG Guanxian, ZHU Menglong, ZHANG Yafei
(Guangxi Road and Bridge Engineering Group Co., Ltd., Nanning 530000, Guangxi, China)
摘要:
为解决隧道超前钻探地质预报在解译过程中存在的主观判断性强、定量数据利用率低、评判标准不统一等问题,通过引入机器学习中的极限梯度提升集成算法模型(extreme gradient boosting,XGBoost),结合钻探数据开展隧道围岩完整程度与围岩级别的双层质量评价研究。一方面,采取数据降噪、等距分割、二级指标计算等数据预处理手段对11 233条原始钻探采样数据进行规律发掘和质量提升;另一方面,结合遗传算法(genetic algorithm,GA)与分类器链(classifier chains,CC)构建GA-CC-XGBoost模型,实现复杂机器学习模型的超参数组合自动寻优以及多标签分类的内在相关性考虑。最终所构建训练集的完整程度与围岩级别2项标签的分类准确率分别为95.91%、97.95%,综合分类准确率为93.88%。经过实际隧道工程应用表明,该模型预测结果满足现场超前钻探地质预报的解译需求。