- CSCD核心中文核心科技核心
- RCCSE(A+)公路运输高质量期刊T1
- Ei CompendexScopusWJCI
- EBSCOPж(AJ)JST
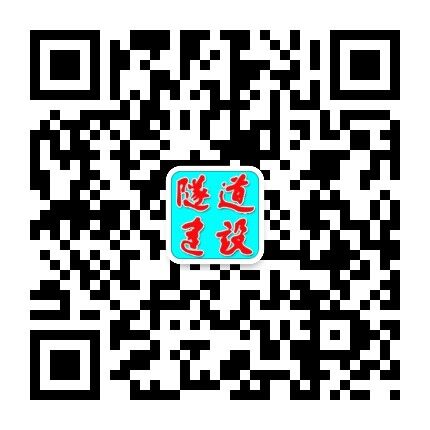
隧道建设(中英文) ›› 2022, Vol. 42 ›› Issue (S2): 36-43.DOI: 10.3973/j.issn.2096-4498.2022.S2.006
王伯芝1,2, 陈文明3, 4, 谢浩1, 2, 丁爽3, 4, 桑运龙3, 4, 刘学增5, *
WANG Bozhi1, 2, CHEN Wenming3, 4, XIE Hao1, 2, DING Shuang3, 4, SANG Yunlong3, 4, LIU Xuezeng5, *
摘要: 掘进参数的有效预测是盾构智能化提升的关键,为建立软硬复合地层下盾构掘进参数的预测模型,提出了地质状况的量化方法。依托济南地铁R1、R2、R3线软硬复合地层下的盾构隧道数据,通过将隧道以及上下方各一个开挖面直径内的地层沿着埋深单元化,分单元统计地层特征参数,建立了地质状况的量化矩阵,进一步基于卷积神经网络自动提取地质量化矩阵的特征,最终实现了掘进参数的预测。研究结果表明: 1)基于地质量化矩阵建立的掘进参数预测模型在训练集、验证集、测试上表现良好; 2)将模型应用于新的地质状况类似的盾构隧道上,刀盘转矩、总推进力、推进速度、刀盘转速的预测值与实际值的变化趋势较为一致,且平均误差在15%之内,预测精度可满足工程需求; 3)对比目前基于加权的地质参数建立的BP神经网络模型,掘进参数的预测精度和稳定性提升明显。