- 中国科学引文数据库(CSCD)来源期刊
- 中文核心期刊中文科技核心期刊
- Scopus RCCSE中国核心学术期刊
- 美国EBSCO数据库 俄罗斯《文摘杂志》
- 《日本科学技术振兴机构数据库(中国)》
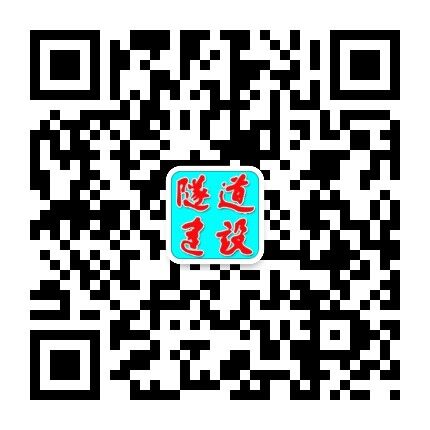
隧道建设(中英文) ›› 2023, Vol. 43 ›› Issue (1): 27-35.DOI: 10.3973/j.issn.2096-4498.2023.01.003
申建红1, 2, 刘树鹏1, *
SHEN Jianhong1, 2, LIU Shupeng1, *
摘要: 为解决不同类型的地铁施工事故关键致因识别,以便于支持事故相关方在风险分析、预防和控制进行决策的问题。在收集国内2011—2021年间发生的202起事故报告数据的基础上,采用树增强朴素贝叶斯(tree augmented naive, TAN)和EM算法,从事故经过、直接原因、间接原因3个角度分别对事故报告进行统计处理、风险指标提取及合并、风险指标筛选、模型图形结构构建、模型参数确定,并采用GENIE软件训练数据建立最终分析模型。贝叶斯模型分析结果表明: 1)通过正向推理明确不同类型事故的关键致险因素,并对各风险因素引发事故的总体影响程度进行重要度排序; 2)通过反向诊断说明所建模型在不同风险因素组合情境下对风险预测的决策支持作用; 3)10折交叉验证证实了模型的有效性。