- 中国科学引文数据库(CSCD)核心期刊
- 中文核心期刊中文科技核心期刊
- Scopus RCCSE中国权威学术期刊
- 美国EBSCO数据库 俄罗斯《文摘杂志》
- 《日本科学技术振兴机构数据库(中国)》
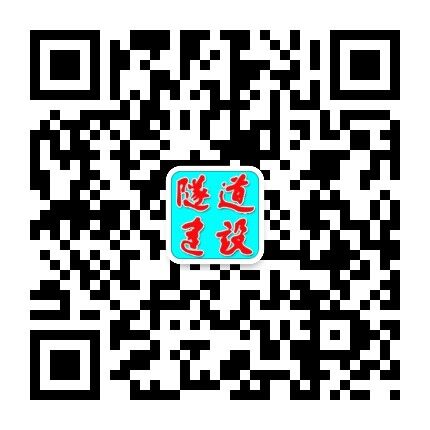
隧道建设(中英文) ›› 2024, Vol. 44 ›› Issue (9): 1752-1761.DOI: 10.3973/j.issn.2096-4498.2024.09.004
丁浩1, 2, 周陈一1, 2, *, 郭鸿雁1, 2, 周云腾1, 2
Knowledge-Data-Driven Analysis Method for Safety Status of Immersed Tube Tunnel Joints: A Case Study of Subsea Immersed Tube Tunnel of Hong Kong-Zhuhai-Macao Bridge
DING Hao1, 2, ZHOU Chenyi1, 2, *, GUO Hongyan1, 2, ZHOU Yunteng1, 2
(1. China Merchants Chongqing Communications Technology Research & Design Institute Co., Ltd., Chongqing 400067, China; 2. National Engineering Research Center for Road Tunnel, Chongqing 400067, China)
摘要: 为解决沉管隧道接头安全状态不断变化且难以直接感知的技术难点,以港珠澳大桥海底沉管隧道为工程背景,通过分析既有监测数据,总结管节接头的变形模式,揭示管节接头张合量与结构温度的强相关性,明确潮位变化对接头剪切变形的显著影响。在此基础上提出一种基于知识-数据驱动的沉管隧道接头变形快速推演方法,通过建立沉管隧道精细化有限元模型,开展海量典型变形模式下的沉管隧道结构力学行为分析,构建沉管隧道变形服役行为数据集; 利用BP神经网络,建立基于仿真接头服役行为特征的沉管隧道接头全断面变形推演模型,实现基于有限实测数据的接头全断面变形快速重构。该方法在港珠澳大桥海底沉管隧道的现场管养中得到成功应用。以2023年台风“苏拉”为例,基于台风登陆过程中接头的局部位移实测数据,推演接头剪力键及止水带关键点位处管节接头的变形情况。结果表明,该沉管隧道接头系统整体受台风影响较小。