- 中国科学引文数据库(CSCD)来源期刊
- 中文核心期刊中文科技核心期刊
- Scopus RCCSE中国核心学术期刊
- 美国EBSCO数据库 俄罗斯《文摘杂志》
- 《日本科学技术振兴机构数据库(中国)》
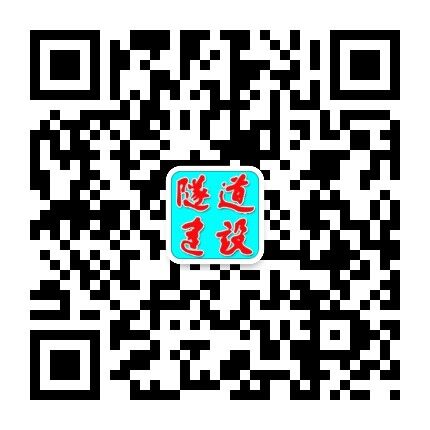
隧道建设(中英文) ›› 2020, Vol. 40 ›› Issue (S1): 247-253.DOI: 10.3973/j.issn.2096-4498.2020.S1.031
张钧博, 何川, 严健*, 吴枋胤, 蒙伟
ZHANG Junbo, HE Chuan, YAN Jian*, WU Fangyin, MENG Wei
摘要: 为解决机器学习算法在样本较少时,所得岩爆烈度等级的预测结果存在可靠性不足的问题,采用一种基于交叉验证的XGBoost算法,并讨论其适用性。先选取岩石单轴抗压强度σc、单轴抗拉强度σt、洞室围岩最大切应力σθ、岩石弹性变形指数Wet以及岩体完整性系数KV等5个评价指标; 再以国内外岩爆实例数据为样本,通过多次交叉验证计算XGBoost算法岩爆预测准确率,与支持向量机算法、随机森林算法所得准确率比较; 最后对评价指标重要性进行分析。结果表明: 1)在样本较少时,样本划分和排序的随机性对预测结果影响较大,通过多次交叉验证求取预测结果平均值,可提高结果可靠性; 2)评价指标中KV与σθ重要性最大,σc重要性最小; 3)XGBoost算法具有较高的预测准确率,在岩爆烈度分级预测中具有一定适用性。
中图分类号: