- CSCD核心中文核心科技核心
- RCCSE(A+)公路运输高质量期刊T1
- Ei CompendexScopusWJCI
- EBSCOPж(AJ)JST
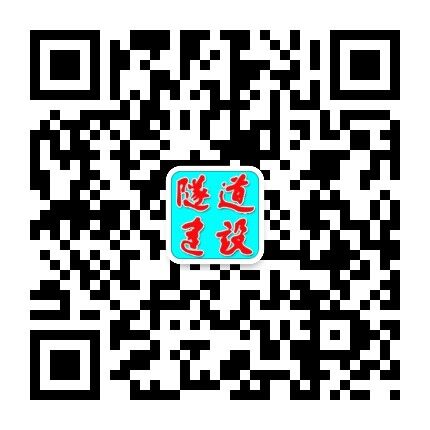
隧道建设(中英文) ›› 2022, Vol. 42 ›› Issue (11): 1879-1888.DOI: 10.3973/j.issn.2096-4498.2022.11.007
赵雪, 顾伟红*
Adaptability Evaluation of Tunnel Bored by TunnelBoring Machine Based on Grey Relational AnalysisSparrow Search AlgorithmElman Neural Network
ZHAO Xue, GU Weihong*
摘要: 为准确评价隧洞施工TBM掘进适应性,保障TBM安全、高效施工,提出一种基于灰色关联分析(GRA)与麻雀搜索算法(SSA)优化Elman神经网络的TBM掘进适应性预测模型。首先,从地质条件、掘进参数、不良地质、施工组织4个方面综合考虑,初步选取13个主要影响因素,建立隧洞TBM掘进适应性评价指标体系; 然后,利用GRA分析指标与掘进适应性间的关联性,引入SSA优化Elman神经网络,提高模型性能,并采用留一交叉验证法验证模型的准确性及可靠性,使得模型最接近原始数据分布特征; 最后,结合北疆水利工程某标段中待测样本对模型预测效果进行验证,同时与Elman、PSO-Elman、BP神经网络模型预测结果及现场实际结果对比分析。结果表明: SSA-Elman模型预测结果与实际工程结果吻合度较高,该模型能够正确、有效地对TBM掘进适应性进行预测评价,且具有合理性和可操作性,可为隧洞TBM适应性评价提供一种新方法。