- 中国科学引文数据库(CSCD)来源期刊
- 中文核心期刊中文科技核心期刊
- Scopus RCCSE中国核心学术期刊
- 美国EBSCO数据库 俄罗斯《文摘杂志》
- 《日本科学技术振兴机构数据库(中国)》
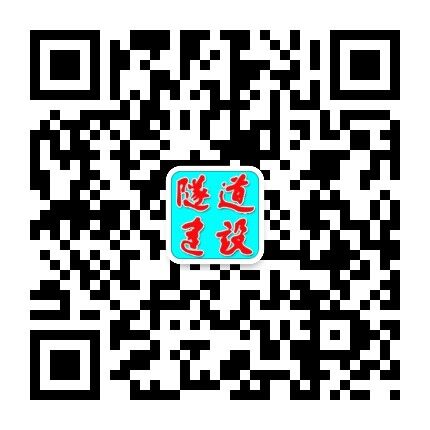
隧道建设(中英文) ›› 2017, Vol. 37 ›› Issue (9): 1105-1113.DOI: 10.3973/j.issn.1672-741X.2017.09.007
王兴科, 王娟
WANG Xingke, WANG Juan[
摘要:
为解决基坑变形预测精度低的问题,采用小波去噪分离基坑变形的趋势项及误差项序列,并利用多种优化的支持向量机对趋势项序列进行预测,采用混沌BP神经网络对误差项序列进行预测,将两者预测结果进行叠加即得到变形预测值,且可根据后期监测数据的更新,实时增加数据信息,达到跟踪预测的目的。经过3个实例检验,得出小波函数的去噪效果相对较优,且预测结果的相对误差均值均小于2%,验证了优化支持向量机-混沌BP神经网络模型的有效性,且该模型具有预测精度高、 适用性强等优点,对掌握基坑变形的发展趋势及评价基坑的稳定性具有重要意义。
中图分类号: