- 中国科学引文数据库(CSCD)来源期刊
- 中文核心期刊中文科技核心期刊
- Scopus RCCSE中国核心学术期刊
- 美国EBSCO数据库 俄罗斯《文摘杂志》
- 《日本科学技术振兴机构数据库(中国)》
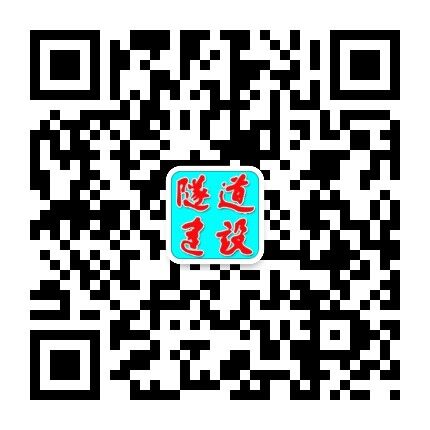
隧道建设(中英文) ›› 2019, Vol. 39 ›› Issue (S1): 220-226.DOI: 10.3973/j.issn.2096-4498.2019.S1.031
赵俊杰1, 贾斌2, 张东2, 曹明星1, 李德武1, *
ZHAO Junjie1, JIA Bin2, ZHANG Dong2, CAO Mingxing1, LI Dewu1, *
摘要:
为研究高寒地区设置中心深埋水沟单线铁路隧道的围岩力学参数问题,以兰新铁路新建博州支线阿拉套山隧道为工程背景,基于FLAC3D数值模拟软件联合MATLAB中的神经网络工具箱构建BP神经网络算法,建立隧道开挖位移正演和反演模型,对围岩物理力学参数作反演分析。通过对中心水沟开挖前的拱顶和拱腰监测数据做拟合分析,发现隧道变形已趋于稳定,反演过程不需考虑中心水沟开挖对围岩的二次扰动。以水沟开挖前的拱顶沉降值和拱腰收敛值作为输入函数,以围岩的体积弹性模量K、剪切弹性模量G、黏聚力c、内摩擦角φ、重度γ作为输出函数训练神经网络模型,利用训练好的模型进行所需参数的反演分析。将反演参数代入FLAC3D正演模型计算后,提取中心水沟开挖前的拱顶沉降值和拱腰收敛值,与中心深埋水沟开挖前的实际监控量测值相比较为接近。结果证明,围岩物理力学参数的反演较为合理,对于变形的预测较为准确,可为隧道后期工程的施工和优化设计提供参考。
中图分类号: