- CSCD核心中文核心科技核心
- RCCSE(A+)公路运输高质量期刊T1
- Ei CompendexScopusWJCI
- EBSCOPж(AJ)JST
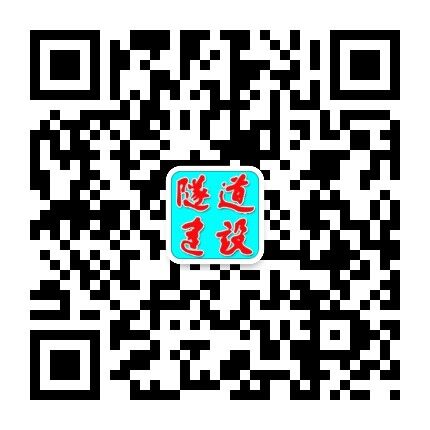
隧道建设(中英文) ›› 2018, Vol. 38 ›› Issue (6): 941-947.DOI: 10.3973/j.issn.2096-4498.2018.06.008
陈艳茹
CHEN Yanru
摘要:
为解决传统智能算法网络结构参数复杂、运算速度慢等问题,基于遗传算法和极限学习机构建基坑变形的新型优化智能预测模型。先利用皮尔逊相关系数评价不同影响因素与基坑沉降变形之间的相关性,以确定极限学习机的输入层; 再采用试算法确定最优激励函数和隐层节点数,并将遗传算法和极限学习机耦合,利用遗传算法优化极限学习机的初始权值和阈值,以提高预测精度。经实例检验表明: 1)开挖时间、开挖深度、土体抗剪参数及重度均与基坑沉降变形显著相关,为构建极限学习机输入层提供了依据; 2)在预测过程中,激励函数和隐层节点数对极限学习机的预测效果具有一定的影响,以Sigmiod型激励函数和13个隐层节点数的预测效果为最优; 3)通过遗传算法的优化,能进一步提高预测精度,验证了遗传算法的优化能力和有效性。预测模型在不同工况下的预测结果均较优,说明该模型具有较高的稳定性和可靠性。
中图分类号: