- CSCD核心中文核心科技核心
- RCCSE(A+)公路运输高质量期刊T1
- Ei CompendexScopusWJCI
- EBSCOPж(AJ)JST
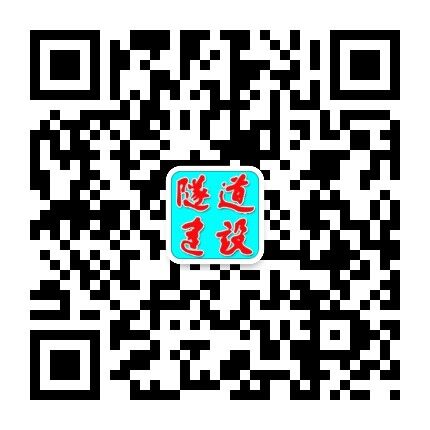
隧道建设(中英文) ›› 2021, Vol. 41 ›› Issue (10): 1733-1739.DOI: 10.3973/j.issn.2096-4498.2021.10.012
刘锦1, 李峰辉2, 刘秀秀3
(1. 陕西铁路工程职业技术学院城轨工程学院, 陕西 渭南 714000;2. 郑州市交通规划勘察设计研究院, 河南 郑州 450000; 3. 曲阜远东职业技术学院, 山东 济宁 273100)
Optimized Genetic AlgorithmBack Propagation Neural Network Model and Its Application in Foundation Pit Deformation Prediction
LIU Jin1, LI Fenghui2, LIU Xiuxiu3
(1. Urban Railway Engineering Department, Shannxi Railway Institute, Weinan 714000, Shannxi, China; 2. Zhengzhou Communications Planning, Survey and Design Institute, Zhengzhou 450000, Henan, China; 3. Qufu Fareast Vocational and Technical College, Jining 273100, Shandong, China)
摘要: 针对现有GA-BP神经网络预测模型在训练样本预处理和隐含层结构设计方面的不足,通过相关系数回归分析确定最佳归一化区间,利用统计学原理推导得到隐含层神经元个数的解析式,并提出与其相适应的最佳单隐含层神经元个数的取值范围。结果表明: 1)经相关系数回归分析确定训练样本预处理的最佳归一化区间为[0.05,0.95]; 2)通过统计对比和反复试算,得到单隐含层结构最佳神经元个数区间为[4,7],双隐层更适用于神经元个数较多的情况(>4); 3)“新陈代谢”方式选取训练样本可显著降低基坑变形的“时空效应”和人为因素干扰; 4)构建“4—2(7,8)—1”型GA-BP神经网络模型,对不同深度基坑进行水平位移预测,精度评价指标表明优化的GA-BP神经网络模型预测效果良好,对工程开展具有参考价值,经多模型对比可知模型优化效果良好。
中图分类号: