- CSCD核心中文核心科技核心
- RCCSE(A+)公路运输高质量期刊T1
- Ei CompendexScopusWJCI
- EBSCOPж(AJ)JST
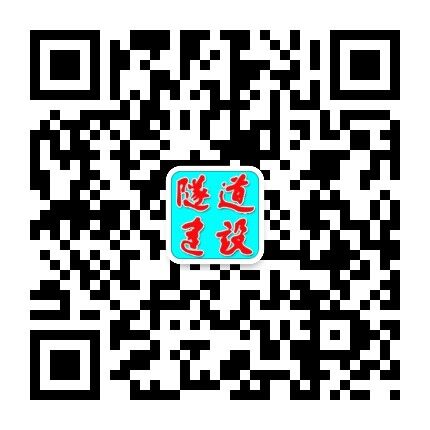
隧道建设(中英文) ›› 2021, Vol. 41 ›› Issue (12): 2122-2132.DOI: 10.3973/j.issn.2096-4498.2021.12.013
荣明1, 陈英杰1, 黄超2, *, 王大川1, 原俊峰3
(1. 新疆农业大学水利与土木工程学院, 新疆 乌鲁木齐 830052; 2. 新疆工程学院安全科学与工程学院, 新疆 乌鲁木齐 830052; 3. 新疆兵团水利水电工程集团有限公司, 新疆 乌鲁木齐 830000)
Research and Application of Personnel Safety Detection Technology for Tunnels Based on One-Class Support Vector Machine
RONG Ming1, CHEN Yingjie1, HUANG Chao2, *, WANG Dachuan1, YUAN Junfeng3
(1. College of Hydraulic and Civil Engineering, Xinjiang Agricultural University, Urumqi 830052, Xinjiang, China;
2. College of Safety Science and Engineering, Xinjiang Institute of Engineering, Urumqi 830052, Xinjiang, China;
3. Xinjiang Bingtuan Water Conservancy and Hydropower Construction Engineering Group Co., Ltd., Urumqi 830000, Xinjiang, China)
摘要: 隧道人员安全状态的判断主要通过收集施工人员的体征和洞内环境数据,对异常状态的预警通常需要专业工作人员在短时间内迅速作出判断,运维成本高且工作效率较低。针对此现象,提出一种基于单分类支持向量机的人员安全状态检测以及预警模型。首先,通过在现场部署传感器设备,获取实际隧道施工场景安全状态下的数据,并构建OCSVM模型进行异常状态预测;接着,保留模型进行预警状态测试,从工程实例中收集相关环境数据以及施工人员体征数据,并进行横向不同参数模型试验和纵向不同预警状态比例数据试验;最后,评估模型对人员信息安全状态判断的性能。试验结果表明,人员安全状态预警准确率达到90%以上。
中图分类号: