- CSCD核心中文核心科技核心
- RCCSE(A+)公路运输高质量期刊T1
- Ei CompendexScopusWJCI
- EBSCOPж(AJ)JST
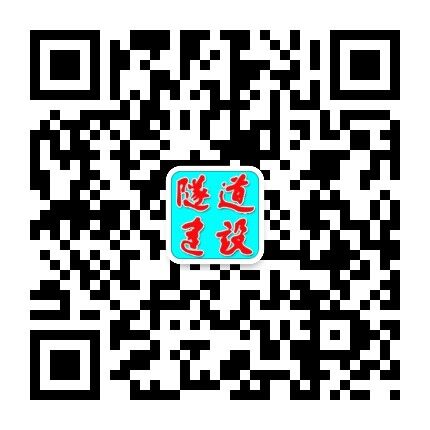
隧道建设(中英文) ›› 2024, Vol. 44 ›› Issue (5): 1012-1028.DOI: 10.3973/j.issn.2096-4498.2024.05.010
张建明1, 2, 3, 侍克斌2, 3, *,
贾运甫1, 任志强4, 巴合特别克·达拉依汗4, 刘昭4
(1. 新疆水利水电勘测设计研究院有限责任公司, 新疆 乌鲁木齐 830000; 2. 新疆农业大学水利与土木工程学院, 新疆 乌鲁木齐 830052; 3. 新疆水利工程安全与水灾害防治重点实验室,新疆 乌鲁木齐 830052; 4. 新疆水发建设集团有限公司, 新疆 乌鲁木齐 830063)
Shapley Additive Explanations Interpretation Model for Penetration Rate Prediction of Tunnel Boring Machines Based on Variational
Mode Decomposition and Weighted Random Forest
ZHANG Jianming1, 2, 3,
SHI Kebin2, 3, *, JIA Yunfu1, REN Zhiqiang4, BAHETEBIEKE Dalayihan4, LIU Zhao
(1. Xinjiang Water Conservancy and Hydropower Survey Design Institute Co., Ltd., Urumqi 830000, Xinjiang, China; 2. College of Water Conservancy and Civil Engineering, Xinjiang Agricultural University, Urumqi 830052, Xinjiang, China; 3. Xinjiang Key Laboratory of Hydraulic Engineering Security and Water Disaster Prevention, Urumqi 830052, Xinjiang, China; 4. Xinjiang Shuifa Construction Group Co., Ltd., Urumqi 830063, Xinjiang, China)
摘要: 为较准确地实现TBM掘进速度(PR)的预测,构建一套基于加权随机森林(RF)结合变分模态分解(VMD)的集成学习预测模型。模型建立过程中,通过收集来自KS隧洞与兰州水源地输水隧洞中涵盖不同岩性下的数据,利用VMD对数据进行4次模态分解,在保留数据特性的同时去除最高频噪音;采用SHAP对未加权传统RF从模型贡献角度进行特征度量,以此实现未加权传统RF加权,并使用RFECV与网格搜索对加权RF进行特征遴选、超参数优化;通过实际工程对模型的性能进行验证,基于SHAP理论对模型从全局与局部进行解释。结果表明: 1)所建模型预测精度较高,其在测试集上的均方根误差(MSE)、平均绝对误差(MAE)与决定系数(R2)分别为0.064 9 (m/h)2、0.187 5 m/h、0.925 4。2)在实际工程的验证中,模型取得了MSE=0.050 3 (m/h)2、MAE=0.161 3 m/h、R2=0.950 5的性能表现,精度理想,且性能均高于常用的深度神经网络、支持向量回归、未加权传统RF。3)经过VMD处理可有效提升PR的预测精度,处理后的模型在测试集上MSE、MAE、R2分别提升了82.50%、59.00%、33.25%。4)岩石单轴抗压强度是精准预测PR时最重要的因素,地质参数在预测中的交互性明显优于掘进参数。预测分析重要洞段的PR时,需结合全局与局部2个角度进行综合分析。