- CSCD核心中文核心科技核心
- RCCSE(A+)公路运输高质量期刊T1
- Ei CompendexScopusWJCI
- EBSCOPж(AJ)JST
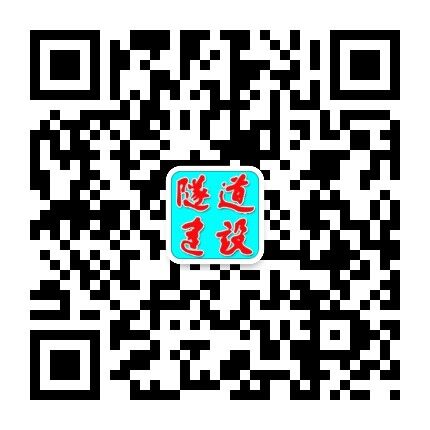
隧道建设(中英文) ›› 2024, Vol. 44 ›› Issue (10): 1961-1970.DOI: 10.3973/j.issn.2096-4498.2024.10.005
鲍艳1, 梅崇斌1, 徐鹏宇2, 孙哲1, 温玉成1
BAO Yan1, MEI Chongbin1, XU Pengyu2, SUN Zhe1, WEN Yucheng1
(1. College of Architecture and Civil Engineering, Beijing University of Technology, Beijing 100124, China; 2. Beijing Urban Construction Exploration & Surveying Design Research Institute Co., Ltd., Beijing 100101, China)
摘要: 为解决地铁隧道裂缝智能识别困难,尤其针对地铁隧道裂缝检测天窗时间短、人工检测效率低、裂缝识别特征不明显、隧道内干扰物较多等问题,提出一种基于YOLOv8的改进算法——M-YOLO(Modified-YOLO),高效智能识别隧道裂缝。M-YOLO 算法运用全维度动态卷积取代传统卷积模块,能显著提高检测的准确性,避免模型参数膨胀的问题; 引入 C2fGC 模块对网络结构进行改进,构建新的特征提取与降维机制,增强高层次特征表示; 整合CBAM 注意力机制模块,强化对裂缝区域图像的特征学习与提取,减弱背景干扰,进而有效提升检测精度; 引入 WIOU 损失函数来调节几何因素的惩罚程度,提高模型的泛化能力,在低质量数据样本下的表现更为出色。试验结果表明,在地铁隧道裂缝识别的真实样本中,M-YOLO 算法的 PmAP(平均精度均值)高达 83.0%,较原模型提高了15.7%。