- 中国科学引文数据库(CSCD)来源期刊
- 中文核心期刊中文科技核心期刊
- Scopus RCCSE中国核心学术期刊
- 美国EBSCO数据库 俄罗斯《文摘杂志》
- 《日本科学技术振兴机构数据库(中国)》
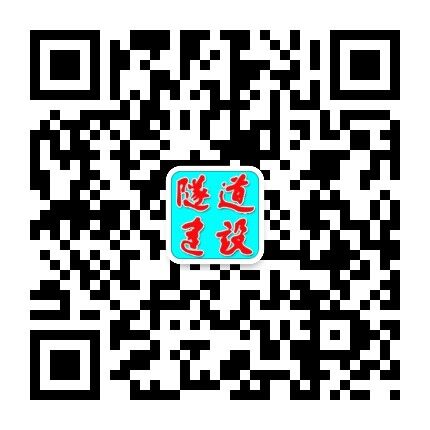
隧道建设(中英文) ›› 2021, Vol. 41 ›› Issue (S2): 261-267.DOI: 10.3973/j.issn.2096-4498.2021.S2.033
何嵘国1, 张孝勇2, 汪旭1,*, 赵子越1, 安顺彪1
HE Rongguo1, ZHANG Xiaoyong2, WANG Xu1, *, ZHAO Ziyue1, AN Shunbiao1
摘要: 针对现有数据处理方法对离散型和波动性较大的监控量测数据进行预测分析时存在不足的问题,基于深度学习的循环神经网络算法(recurrent neural network, RNN),对地铁隧道施工监控量测数据进行建模分析,构建长短期记忆网络(long shortterm memory, LSTM)模型,对某隧道工程的拱顶下沉数据进行分析预测,并与目前较为常用的灰色理论预测法、BP神经网络、非线性自回归(nonautoregressive, NAR)神经网络及支持向量回归(support vactor regression, SVR)预测方法的预测效果进行对比。结果表明: 1)循环神经网络对时间序列具有敏感性,在对监控量测数据的预测上表现出了较强的优势,可以实现对监控量测数据的精确预测,无论是对波动程度较小的数据还是对波动程度较大的数据,其预测效果要优于目前常用的方法。2)基于深度学习的循环神经网络算法具有收敛速度快、稳定性好、预测精度高的优点。