- CSCD核心中文核心科技核心
- RCCSE(A+)公路运输高质量期刊T1
- Ei CompendexScopusWJCI
- EBSCOPж(AJ)JST
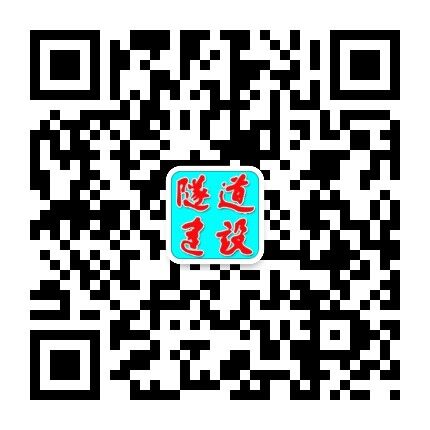
隧道建设(中英文) ›› 2025, Vol. 45 ›› Issue (4): 677-686.DOI: 10.3973/j.issn.2096-4498.2025.04.002
洪开荣1, 2, 3, 王凯1, 2, 3,*, 李站国1, 2, 3, 陈瑞祥1, 2, 3, 李凤远1, 2, 3, 陈桥1, 2, 3,秦银平1, 2, 3
HONG Kairong1, 2, 3, WANG Kai1, 2, 3, *, LI Zhanguo1, 2, 3, CHEN Ruixiang1, 2, 3, LI Fengyuan1, 2, 3, CHEN Qiao1, 2, 3, QIN Yinping1, 2, 3
摘要: 盾构岩机作用载荷(盾构推力、刀盘转矩)对于装备设计、现场施工等尤为重要,但现有理论或统计模型参数多、计算繁杂,为提升盾构数字化设计、数字孪生水平,高效精准地进行载荷赋值仍需探索。针对已有机器学习算法重视各地层比例与力学参数等,却忽视地层空间位置关系的现状,融合图像、数据序列进行多特征信息表征,提出一种基于卷积神经网络(CNN)、双向长短期记忆(BiLSTM)与注意力机制(Attention)的数据驱动型超大直径泥水盾构岩机作用载荷预测方法。首先,利用K-means算法对地质纵剖面图进行地层信息提取并切片,对切片图像进行CNN特征提取,结合装备运行数据形成多特征融合数据集;其次,采用BiLSTM网络提取输入特征的双向时序信息,以注意力机制为后续特征进行权重再分配;最后,利用灰狼优化算法(gray wolf optimizer,GWO)对深度学习网络超参数寻优。研究结果表明: GWO-CNN-BiLSTM-Attention模型相比BP(BP 神经网络)、PSO-BP(粒子群优化的BP神经网络)、LSTM(长短期记忆网络)对盾构推力、刀盘转矩的预测效果更佳,R2均超过0.9,平均相对误差<1%;由于融入了地层位置信息,模型对岩机作用载荷问题具有更强的适用性。