- CSCD核心中文核心科技核心
- RCCSE(A+)公路运输高质量期刊T1
- Ei CompendexScopusWJCI
- EBSCOPж(AJ)JST
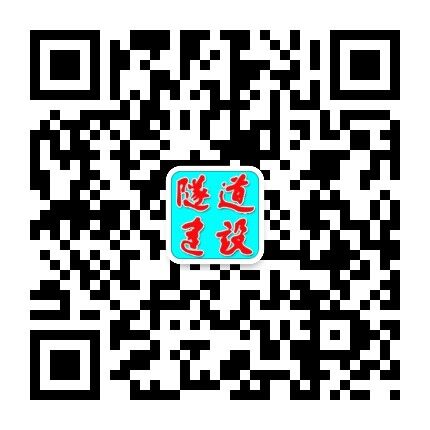
隧道建设(中英文) ›› 2023, Vol. 43 ›› Issue (9): 1485-1491.DOI: 10.3973/j.issn.2096-4498.2023.09.005
乔金丽1, 陈帅1, 陈小强2, *, 郝刚立3, 胡建帮1, 孙永涛1
QIAO Jinli1, CHEN Shuai1, CHEN Xiaoqiang2, *, HAO Gangli3, HU Jianbang1, SUN Yongtao1
摘要: 为解决TBM在掘进过程中因未知的地质条件导致的机器卡顿、停机及岩体坍塌等问题,以围岩的岩体完整性指数、单轴抗压强度、内摩擦角、黏聚力、变形模量、泊松比、坚固性系数和弹性抗力系数等主要力学参数为依据,利用完全自适应噪声集合经验模态分解(CEEMDAN)和时间卷积神经网络(TCN)结合的方式,遵循“先分解再重构”的原则,提出一种基于CEEMDAN-TCN组合模型的围岩等级预测方法,对隧道掌子面处的围岩等级进行预测评价。结果表明: 1)基于CEEMDAN-TCN组合模型的围岩等级预测值与真实值之间的均方误差一般小于0.07,均方根误差一般小于1.67,平均绝对百分比误差一般小于0.45,平均绝对误差一般小于0.14,拟合系数为95.2%; 2)CEEMDAN-TCN组合模型具有误差小、拟合效果佳和实用性高等优点,能准确地预测隧道掌子面处的围岩等级,实现围岩类别的智能分类,对实现TBM高效掘进和风险预警有着重要意义。