- 中国科学引文数据库(CSCD)来源期刊
- 中文核心期刊中文科技核心期刊
- Scopus RCCSE中国核心学术期刊
- 美国EBSCO数据库 俄罗斯《文摘杂志》
- 《日本科学技术振兴机构数据库(中国)》
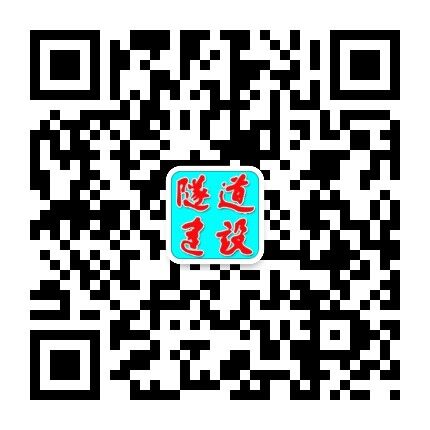
隧道建设(中英文) ›› 2020, Vol. 40 ›› Issue (S1): 107-114.DOI: 10.3973/j.issn.2096-4498.2020.S1.014
复杂地层中盾构掘进速度的调控分析——以新建铁路横琴至珠海机场段HJZQ-2标隧道工程为例
朱小藻
ZHU Xiaozao
摘要: 为解决复杂软弱地层中土压平衡盾构掘进速度难以用理论方法预测调控的问题,基于皮尔森相关系数分析了掘进参数与掘进速度的相关性,并提出盾构掘进速度的深度学习预测模型。模型运用粒子群优化算法对BP神经网络的权值与偏置值进行优化,以克服基于梯度下降算法的传统BP神经网络易陷入局部最小值和预测误差大等缺点,预测模型将地质参数与掘进参数作为输入值,盾构掘进速度作为输出值(预测值)。以新建铁路横琴至珠海机场段HJZQ-2标隧道工程为依托,基于贯入度与掘进速度的相关性最高且呈正相关的分析结果,采用监测数据对模型进行训练,利用训练后的深度学习模型对掘进速度进行预测分析。结果显示,具有2层隐藏层的深度学习PSO-BP模型的预测误差基本控制在±4 mm/min(误差在10%以内),满足实际工程要求,从而验证了模型的有效性与适用性。