- CSCD核心中文核心科技核心
- RCCSE(A+)公路运输高质量期刊T1
- Ei CompendexScopusWJCI
- EBSCOPж(AJ)JST
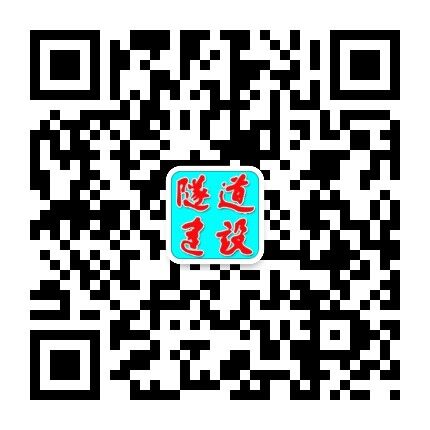
隧道建设(中英文) ›› 2013, Vol. 33 ›› Issue (11): 903-907.DOI: 10.3973/j.issn.1672-741X.2013.11.002
周先仓
ZHOU Xiancang
摘要:
围岩分级准确与否直接关系到隧道的施工安全和工程造价。针对现阶段围岩分级方法存在的主要问题,结合宁绩高速公路隧道群施工期围岩定级实践,以国标BQ分级为基准,在大量现场测试和室内试验的基础上,引入径向基函数神经网络,并以分级结果作为遗传—径向基函数神经网络的训练样本,建立了隧道围岩分级的遗传—径向基函数神经网络模型。应用实例表明,该模型分级结果与现场勘测基本一致,为隧道围岩分级提供了一种新方法。