- CSCD核心中文核心科技核心
- RCCSE(A+)公路运输高质量期刊T1
- Ei CompendexScopusWJCI
- EBSCOPж(AJ)JST
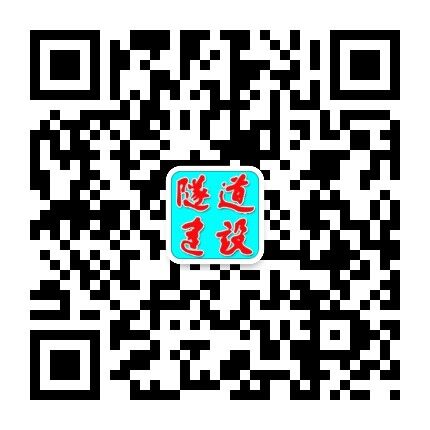
隧道建设(中英文) ›› 2018, Vol. 38 ›› Issue (6): 934-940.DOI: 10.3973/j.issn.2096-4498.2018.06.007
马琳
MA Lin
摘要:
为提高基坑变形预测精度及稳定性,首先,利用遗传算法优化BP神经网络的结构参数,再将参数优化后的BP神经网络与灰色模型结合,构建出GA-BP神经网络模型,并利用该模型实现基坑变形序列的初步预测; 其次,基于残差序列的混沌特性,再利用混沌理论进行残差优化,进一步构建考虑混沌特性优化的GA-BP神经网络模型; 最后,将SR检验引入到基坑变形趋势判断中,以检验预测结果的准确性。实例检验表明: 通过遗传算法及混沌理论的递进优化,能逐步提高预测精度,验证文章预测模型的有效性,且预测结果与SR检验结果的一致性较好,说明该预测模型的可信度高。
中图分类号: