- 中国科学引文数据库(CSCD)来源期刊
- 中文核心期刊中文科技核心期刊
- Scopus RCCSE中国核心学术期刊
- 美国EBSCO数据库 俄罗斯《文摘杂志》
- 《日本科学技术振兴机构数据库(中国)》
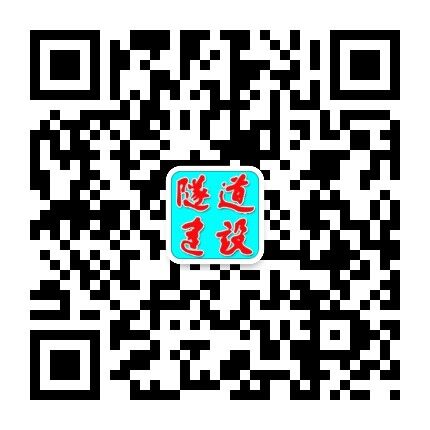
隧道建设(中英文) ›› 2021, Vol. 41 ›› Issue (S2): 336-345.DOI: 10.3973/j.issn.2096-4498.2021.S2.043
白祥瑞1, 戎晓力2, *, 文祝2, 张宁1
BAI Xiangrui1, RONG Xiaoli2, *, WEN Zhu2, ZHANG Ning1
摘要: 为获取更加有效的地表沉降影响因素数据进行地表沉降的机器学习算法预测,基于差分进化算法优化支持向量回归机(DE-SVR)的机器学习方法,结合盾构法隧道施工阶段地表沉降的影响范围,以及该范围内地表沉降影响因素的多元时序数据特征,建立盾构法隧道施工阶段的地表沉降预测方法。以常州轨道交通1号线工程为例,结果表明,与传统的机器学习预测研究方法进行对比,该地表沉降预测方法具有更高的预测精度及更稳定的预测效果。