- CSCD核心中文核心科技核心
- RCCSE(A+)公路运输高质量期刊T1
- Ei CompendexScopusWJCI
- EBSCOPж(AJ)JST
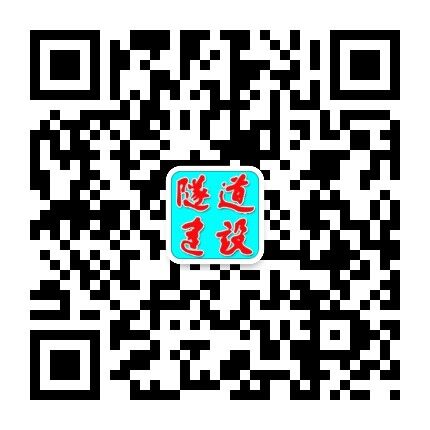
隧道建设(中英文) ›› 2024, Vol. 44 ›› Issue (4): 673-681.DOI: 10.3973/j.issn.2096-4498.2024.04.005
毛勤平1, 刘军成1, 杨晓秋2, 3, *, 刘学增4, 桑运龙2, 3
MAO Qinping1, LIU Juncheng1, YANG Xiaoqiu2, 3, *, LIU Xuezeng4, SANG Yunlong2, 3
摘要: 针对围岩风化程度判定存在主观性强、准确率低的问题,开展基于图像识别和机器学习的围岩风化程度定量化判别技术研究。依托大广复线扩容工程搜集隧道施工期间掌子面的图像,采用数字图像处理技术对掌子面图像颜色信息、表面纹理信息、节理信息进行自动化提取。基于提取的指标参数,借助机器学习方法进行指标筛选,并建立围岩风化程度预测模型。通过研究可以得到: 1)对预测模型准确率贡献度由大到小的指标分别为颜色、完整性和纹理,各单一指标预测模型准确率分别为71.91%、70.78%、47.19%; 2)多指标组合模型相较单一指标模型判定准确率更高,采用颜色、纹理、完整性3个指标的组合模型,准确率达到86.52%; 3)对13个二级指标进行特征筛选,剔除5个贡献度较低的纹理特征指标,采用剩余的8个关键指标构建预测模型,预测精度仍能达到85%,满足工程需求。研究结果表明: 掌子面图像的颜色、纹理、完整性信息能够有效表征围岩风化程度,建立在这些指标之上的机器学习预测模型能够稳定有效地对掌子面的风化程度进行判定。