- CSCD核心中文核心科技核心
- RCCSE(A+)公路运输高质量期刊T1
- Ei CompendexScopusWJCI
- EBSCOPж(AJ)JST
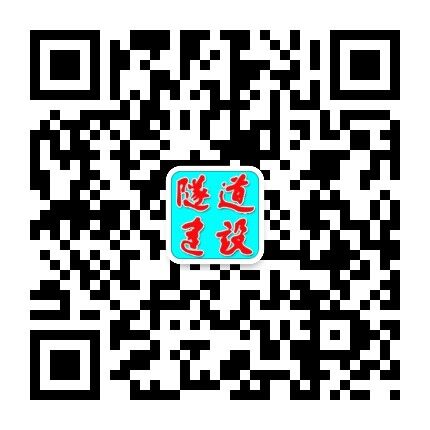
隧道建设(中英文) ›› 2024, Vol. 44 ›› Issue (5): 1056-1067.DOI: 10.3973/j.issn.2096-4498.2024.05.013
向露露1, 2, 童建军1, 2, *, 王明年1, 2, 苗兴旺1, 2, 叶沛1, 2
(1. 西南交通大学 交通隧道工程教育部重点实验室, 四川 成都 610031;2. 西南交通大学土木工程学院, 四川 成都 610031)
XIANG Lulu1, 2, TONG Jianjun1, 2, *, WANG Mingnian1, 2, MIAO Xingwang1, 2, YE Pei1, 2
摘要: 为研究综合高效的隧道掌子面岩性智能分类方法,首先,通过收集高铁沿线施工隧道高清掌子面照片、地质素描图及工程地质说明,筛选并统计出灰岩、泥岩、砂岩、玄武岩4种岩性,在此基础上,采用图像增强扩充样本数量并构建岩性样本集; 然后,基于上述样本集分别构建ResNet50V2岩性分类迁移模型及VIT岩性分类模型,对比二者岩性分类效果,并采用Stacking方法集成2种模型的分类特点; 最后,通过对比3种元学习器(逻辑回归、支持向量机、决策树)对2种模型的集成融合效果来选取最适用的元学习器。结果表明: 采用逻辑回归集成ResNet50V2及VIT所构建的集成模型对岩性的分类效果最好,能充分融合掌子面岩性的全、局部特征来进行分类,模型准确率达到93.8%。