- 中国科学引文数据库(CSCD)来源期刊
- 中文核心期刊中文科技核心期刊
- Scopus RCCSE中国核心学术期刊
- 美国EBSCO数据库 俄罗斯《文摘杂志》
- 《日本科学技术振兴机构数据库(中国)》
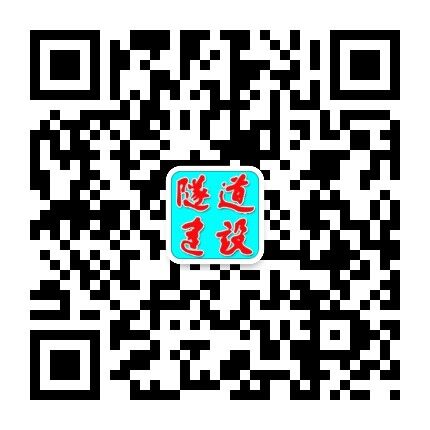
隧道建设(中英文) ›› 2023, Vol. 43 ›› Issue (3): 486-495.DOI: 10.3973/j.issn.2096-4498.2023.03.013
盾构法隧道施工地表变形阶段控制目标规划及其在盾构自主驾驶中的应用
卢靖1, 2, 周文波3, 胡珉2, 4
(1. 上海大学机电工程与自动化学院, 上海 200444;2. 上海大学 上海大学-上海城建(集团)建筑产业化研究中心, 上海 200072;
3. 上海隧道工程股份有限公司, 上海 200032; 4. 上海大学悉尼工商学院, 上海 201800)
Stage Target Planning of Surface Deformation in Shield Tunneling and Its Applications in SelfDriving Shield
LU Jing1, 2, ZHOU Wenbo3, HU Min2, 4
(1.School of Mechanical and Electrical Engineering and Automation,Shanghai University,Shanghai 200444,China;2.SHU-SUCG Research Center for Building Industrialization,Shanghai University,Shanghai 200072,China;3.Shanghai Tunnel Engineering Co., Ltd.,Shanghai 200032,China;4.SHU-UTS SILC Business School,Shanghai University,Shanghai 201800,China)
摘要: 为降低阶段地表变形目标设定中对施工人员经验依赖程度,提高盾构施工的安全性和效率,提出一种科学、有效的地表变形阶段目标规划方法。首先,对不同地质条件下各阶段地表变形量与盾构掘进效率和周边环境的关系进行分析; 然后,采用机器学习方法建立盾构掘进效率评估模型和最终地表变形预测模型; 最后,基于预测结果综合考虑推进效率、环境扰动和施工成本,得到工程综合效益评价,并应用粒子群优化算法计算得到各阶段地表变形控制值,以实现最优综合效益。该模型已被内置在“智驭号”盾构自主掘进控制系统中,并应用于南京地铁5号线隧道工程。工程应用结果表明,该模型通过对地表变形阶段控制目标的优化,有效提升了自主驾驶盾构施工的安全性和高效性。