- 中国科学引文数据库(CSCD)来源期刊
- 中文核心期刊中文科技核心期刊
- Scopus RCCSE中国核心学术期刊
- 美国EBSCO数据库 俄罗斯《文摘杂志》
- 《日本科学技术振兴机构数据库(中国)》
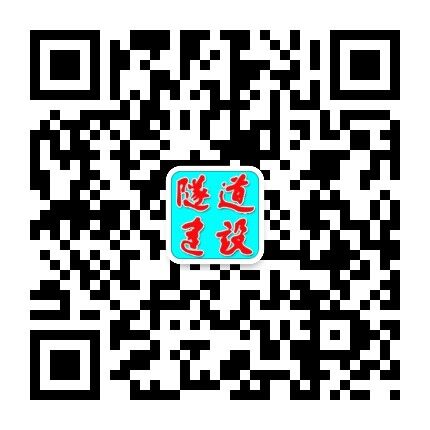
隧道建设(中英文) ›› 2021, Vol. 41 ›› Issue (S1): 11-.DOI: 10.3973/j.issn.2096-4498.2021.S1.002
吴惠明1, 常佳奇2, 3, 李刚1, 张东明2, 3, *, 黄宏伟2, 3
(1. 上海隧道工程股份有限公司, 上海 200032;2. 同济大学地下建筑与工程系, 上海 200092;3. 同济大学岩土及地下工程教育部重点实验室, 上海 200092)
Prediction of Driving Posture and Optimization of Construction Parameters for Shield Based on Support Vector Machine
WU Huiming1, CHANG Jiaqi2, 3, LI Gang1, ZHANG Dongming2, 3, *, HUANG Hongwei2, 3
(1. Shanghai Tunnel Engineering Co., Ltd., Shanghai 200032, China; 2. Department of Geotechnical Engineering College, Tongji University, Shanghai 200092, China;3. Key Laboratory of Geotechnical and Underground Engineering of Ministry of Education, Tongji University,Shanghai 200092, China)
摘要: 为解决盾构司机设定施工参数的标准不明确,且盾构司机的数量难以满足盾构隧道建设需求而引发的盾构姿态难以得到良好控制的问题,需要寻找一种可靠且便于推广使用的施工参数-盾构姿态预测模型。基于盾构施工中的大量数据与机器学习中的支持向量机算法,提出一种预测盾构姿态与优化施工参数的方法。所使用的数据采集自上海地铁14号线新建隧道,时间跨度为299 d,具体包括盾构的施工参数以及相关的地层信息。样本集包括43 030个样本,75%的样本用于训练,25%的样本用于测试。测试结果表明模型的拟合优度达到0.863,在15%的容许误差下准确率达到94.5%,远高于传统的拟合方法。将此模型用于施工参数的优化以实现盾构姿态控制,控制结果表明使用此模型优化的施工参数进行掘进时盾构姿态能够得到良好控制。