ISSN 2096-4498
CN 44-1745/U
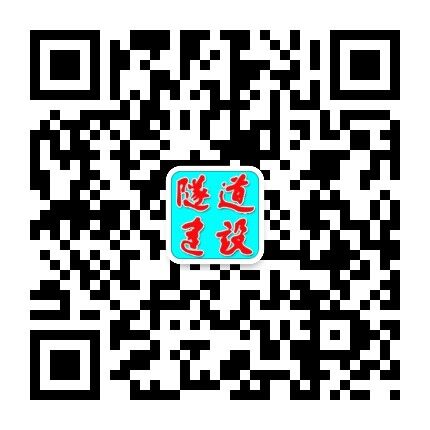
ISSN 2096-4498
CN 44-1745/U
Tunnel Construction ›› 2024, Vol. 44 ›› Issue (10): 1971-1981.DOI: 10.3973/j.issn.2096-4498.2024.10.006
Previous Articles Next Articles
FU Jinyang1, 2, WANG Haoyu1, XIE Jichen1, ZHU Zhiheng1, ZHANG Cong3, WU Jun4