ISSN 2096-4498
CN 44-1745/U
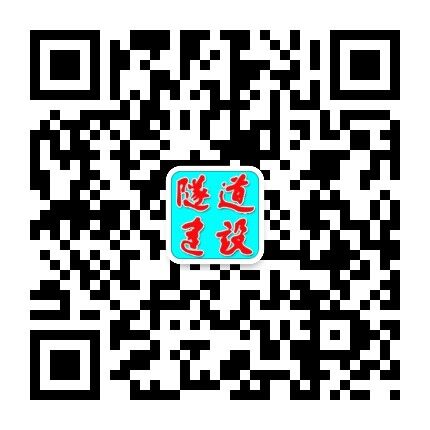
ISSN 2096-4498
CN 44-1745/U
Tunnel Construction ›› 2024, Vol. 44 ›› Issue (11): 2181-2189.DOI: 10.3973/j.issn.2096-4498.2024.11.008
Previous Articles Next Articles
HAN Dong1, ZHANG Mingshu1, TAO Zanxu1, LEI Yu2, *, WU Xianguo2