ISSN 2096-4498
CN 44-1745/U
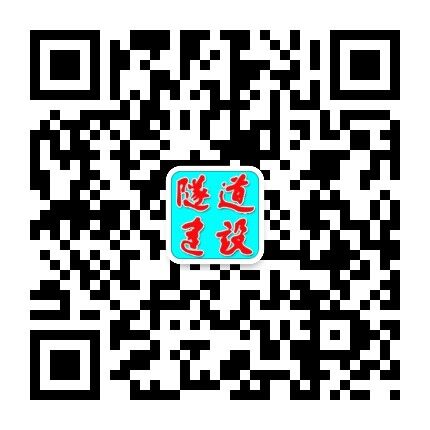
ISSN 2096-4498
CN 44-1745/U
Tunnel Construction ›› 2025, Vol. 45 ›› Issue (1): 139-150.DOI: 10.3973/j.issn.2096-4498.2025.01.011
Previous Articles Next Articles
LIU Zhe1, 2, 3, XU Chao1, 2, 3, XIONG Dongdong1, 2, 3
(1. CCCC Second Harbor Engineering Company Ltd., Wuhan 430040, Hubei, China; 2. Research and Development Center of Transport Industry of Intelligent Manufacturing Technologies for Transport Infrastructure, Wuhan 430040, Hubei, China; 3. Key Laboratory of Large-Span Bridge Construction Technology, Wuhan 430040, Hubei, China)