- CSCD核心中文核心科技核心
- RCCSE(A+)公路运输高质量期刊T1
- Ei CompendexScopusWJCI
- EBSCOPж(AJ)JST
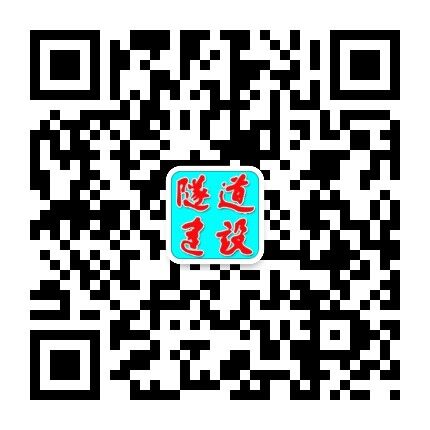
隧道建设(中英文) ›› 2022, Vol. 42 ›› Issue (12): 2048-2063.DOI: 10.3973/j.issn.2096-4498.2022.12.008
陈政, 郭亚林, 郭春*
CHEN Zheng, GUO Yalin, GUO Chun*
摘要:
为解决成都地铁设计和修建过程中碳排放计量问题,以成都地铁18号线6车站7区间为研究对象,采用机器学习算法对成都地铁建设阶段碳排放进行预测研究。基于生命周期评价(life cycle assessment, LCA)框架对地铁车站和盾构区间建筑材料生产阶段、建筑材料运输阶段和现场施工阶段温室气体排放量进行计算,建立基于鲸鱼优化算法(whale optimization algorithm, WOA)的深度极限学习机(deep extreme learning machine, DELM)地铁碳排放预测模型,并与基于风驱动优化(wind driven optimizer, WDO)、灰狼优化(grey wolf optimizer, GWO)、粒子群优化(particle swarm optimizer, PSO)、人工蜂群优化(artificial bee colony, ABC)、多元宇宙优化(multi-verse optimizer, MVO)、原子搜索优化(atom search optimizer,ASO)的深度极限学习机(DELM)和未优化的BP(back propagation neural network)、KELM(kernel extreme learning machine)、DELM算法预测结果进行对比分析。研究得到: 1)WOA-DELM算法预测结果相关一致性为0.757,略高于其他算法; 2)根据WOA-DELM算法对地铁碳排放主要输入指标进行敏感性分析,得到地铁车站碳排放预测的关键影响因素为车站长度和轨面埋深,对应指标碳排放相对变化率分别为30.1%和23.1%。