- CSCD核心中文核心科技核心
- RCCSE(A+)公路运输高质量期刊T1
- Ei CompendexScopusWJCI
- EBSCOPж(AJ)JST
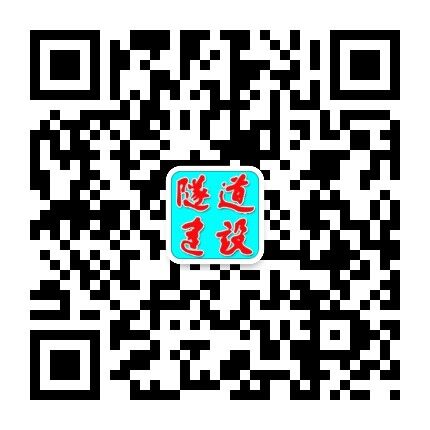
隧道建设(中英文) ›› 2023, Vol. 43 ›› Issue (6): 1035-1044.DOI: 10.3973/j.issn.2096-4498.2023.06.014
吴玲1, 2, 刘建蓓1, *, 单东辉1, 马小龙1, 王元庆3
WU Ling1, 2, LIU Jianbei1, *, SHAN Donghui1, MA Xiaolong1, WANG Yuanqing3
摘要: 为研究高速公路特长隧道环境车道行驶速度特性,采用基于雷达组网技术的实时交通参数获取方法,提取典型特长隧道全路段全样本高精度时序速度数据,在划分隧道入口段、行车段、出口段的基础上,对比分析特长隧道不同路段车道速度分布特性,构建基于时序Transformer框架的特长隧道环境车道行驶速度短时预测模型。结果表明: 1)特长隧道入口段速度均值最低,分布最为离散; 2)在同一路段,各车道85%分位车速统计值呈现依次递减趋势; 3)小客车运行速度整体呈现下降规律,出入口段差值大; 4)货车在特长隧道入口段会降低速度,但小客车实际运行速度较主线限速值高; 5)所构建的速度预测模型准确率可达97.82%,平均绝对误差为1.67 km/h。上述结果表明: 1)暗适应对驾驶人车速控制行为的影响较为显著; 2)主线限速标准对于特长隧道环境并不完全适用,应考虑速度顺适过渡关系,针对不同车型、不同车道提前诱导; 3)所建立的时序Transformer模型适应于高速公路特长隧道环境所有车道短时速度预测。