- CSCD核心中文核心科技核心
- RCCSE(A+)公路运输高质量期刊T1
- Ei CompendexScopusWJCI
- EBSCOPж(AJ)JST
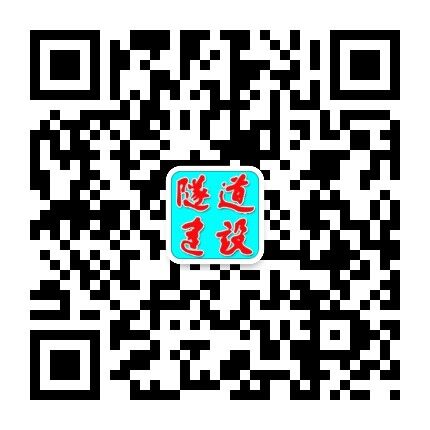
隧道建设(中英文) ›› 2023, Vol. 43 ›› Issue (9): 1492-1500.DOI: 10.3973/j.issn.2096-4498.2023.09.006
曹勇1, 杨川2, 仇文革3, 4, 王先毫5, 白衡斌3, 凌鹏3
(1. 中铁上海设计院集团有限公司, 上海 200070; 2. 皖赣铁路安徽有限责任公司, 安徽 合肥 230000; 3. 成都天佑智隧科技有限公司, 四川 成都 610031; 4. 西南交通大学 交通隧道工程教育部重点实验室,四川 成都 610031; 5. 中铁十局集团第三建筑有限公司, 安徽 合肥 250101)
CAO Yong1, YANG Chuan2, QIU Wenge3, 4, WANG Xianhao5, BAI Hengbin3, LING Peng3
(1. China Railway Shanghai Design Institute Group Corporation Limited, Shanghai 200070, China;2. Anhui Jiangxi Railway Anhui Co., Ltd., Hefei 230000, Anhui, China; 3. Chengdu Tianyou Tunnel Key Company Ltd., Chengdu 610031, Sichuan, China; 4. Key Laboratory of Transportation Tunnel Engineering, the Ministry of Education, Southwest Jiaotong University, Chengdu 610031, Sichuan, China; 5. No.3 Construction Company of China Railway No.10 Engineering Group Co., Ltd., Hefei 250101, Anhui, China)
摘要: 为解决传统人工素描的诸多弊端,提出在掌子面三维几何图像的基础上提取掌子面围岩结构面特征。使用相机和激光雷达采集掌子面围岩数据,建立隧道掌子面三维实景模型;基于围岩结构面法向量的差异,在三维模型上对围岩结构面进行识别;采用卷积神经网络算法训练软件基于地质工程师地质素描识别围岩节理迹线;将三维模型识别结果与图像识别训练进行结合判识,实现掌子面围岩节理迹线的自动识别;基于自动识别结果得到掌子面围岩的岩体体积节理数Jv,从而实现对围岩完整程度的判识。将该方法应用于池黄铁路黄坑隧道和岭上村隧道进行掌子面围岩完整度分析,结果表明: 该方法可获取高精度的掌子面三维模型,能够对隧道掌子面围岩的完整度进行快速、准确的判识,实现隧道地质信息编录。