- CSCD核心中文核心科技核心
- RCCSE(A+)公路运输高质量期刊T1
- Ei CompendexScopusWJCI
- EBSCOPж(AJ)JST
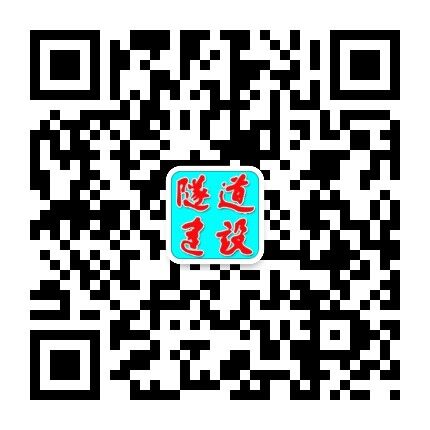
隧道建设(中英文) ›› 2018, Vol. 38 ›› Issue (9): 1456-1462.DOI: 10.3973/j.issn.2096-4498.2018.09.007
赵淑敏
ZHAO Shumin
摘要:
为实现小净距隧道变形影响因素的定量评价,保证隧道变形规律的有效分析,采用数量化理论Ⅲ对小净距隧道的变形影响因素及其耦合性等进行定量分析,并利用极限学习机构建隧道变形预测模型,以验证前者分析结果的准确性。实例分析结果表明: 隧道变形的主导因素有围岩重度、变形模量、泊松比、内摩擦角和黏聚力,重要因素有围岩剪胀角、隧道埋深、喷射混凝土厚度、锚杆长度及隧道间距,而隧道围岩侧压力系数为一般因素; 隧道变形影响因素间存在耦合性,且分析样本4、6、16、18的耦合度高,其余样本的耦合度均为低; 另外,极限学习机的预测结果表明一般影响因素会减弱变形影响因素与变形值间的关联性,对变形规律分析不利,这与变形影响因素分析成果一致,且极限学习机对各监测项目的变形预测效果均较好,验证了该方法在隧道变形预测中的适用性和可靠性。
中图分类号: