- CSCD核心中文核心科技核心
- RCCSE(A+)公路运输高质量期刊T1
- Ei CompendexScopusWJCI
- EBSCOPж(AJ)JST
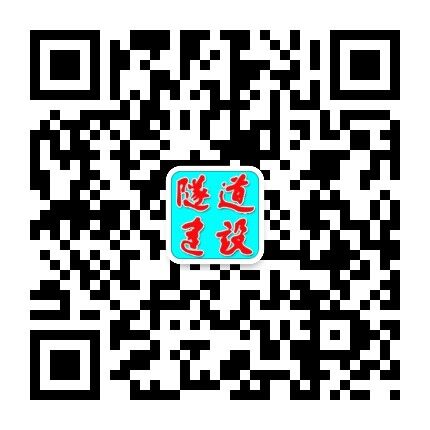
隧道建设(中英文) ›› 2020, Vol. 40 ›› Issue (3): 379-388.DOI: 10.3973/j.issn.2096-4498.2020.03.010
段志伟1, 杜立杰1, ∗, 吕海明2, 王家海2, 刘海东2, 富勇明2
DUAN Zhiwei1, DU Lijie1, ∗, LYU Haiming2, WANG Jiahai2, LIU Haidong2, FU Yongming2
摘要: TBM 围岩可掘性等级实时在线识别和预警对TBM 安全高效以及智能化掘进意义重大,基于新疆EH 隧洞工程直径为7. 0 m 的敞开式TBM 实际掘进数据与地质数据, 通过TBM 掘进性能与施工风险的特征参数指标对围岩进行可掘性分级。在对不同围岩 下区分度较好的掘进参数进行主成分分析之后,获得表征围岩可掘性等级的2 个主成分指标,并在此基础上构建BP 神经网络对围 岩可掘性等级进行识别。同时,为提高模型响应速度,设计了一个MATLAB 程序,从而获得了实用性较强的围岩可掘性等级实时识 别方法。
中图分类号: