- CSCD核心中文核心科技核心
- RCCSE(A+)公路运输高质量期刊T1
- Ei CompendexScopusWJCI
- EBSCOPж(AJ)JST
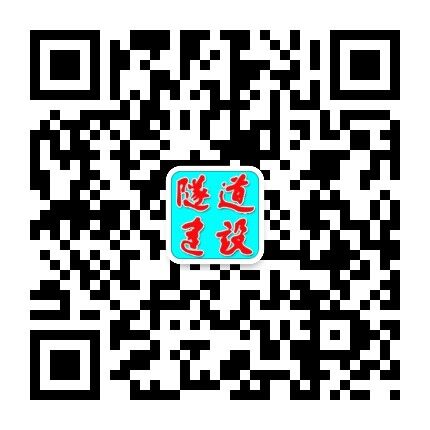
隧道建设(中英文) ›› 2022, Vol. 42 ›› Issue (2): 268-274.DOI: 10.3973/j.issn.2096-4498.2022.02.012
李琛, 骆汉宾, 刘文黎, 柳洋*
Automatic Recognition of Flow Plasticity of Conditioned Soil Based on Improved Faster R-CNN
LI Chen, LUO Hanbin, LIU Wenli, LIU Yang*
(School of Civil Engineering and Hydraulic Engineering, Huazhong University of Science and Technology, Wuhan 430074, Hubei, China)
摘要: 为解决土压平衡盾构掘进过程中存在的操控手检测渣土流塑性费时费力、受主观状态影响较大的问题,提出一种渣土流塑性自动检测方法。首先通过裁剪、恢复、增强等技术对实时监控视频图像进行预处理,构建改良渣土样本数据集,然后建立CSD (conditioned soil detection)网络模型,识别、分类监控视频中渣土流塑性,实现改良渣土流塑性的自动识别。基于实际案例,提出的改进Faster R-CNN网络方法,该方法检测3类改良渣土的平均正确率达91.55%,平均精确率达86.83%,平均召回率达87.42%,检测效果较好,将该模型运用在地铁其他线路中均有较好的检测效果,具有较好的应用推广价值。
中图分类号: