- CSCD核心中文核心科技核心
- RCCSE(A+)公路运输高质量期刊T1
- Ei CompendexScopusWJCI
- EBSCOPж(AJ)JST
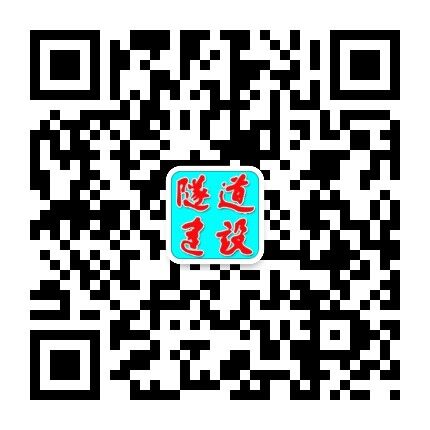
隧道建设(中英文) ›› 2025, Vol. 45 ›› Issue (1): 159-170.DOI: 10.3973/j.issn.2096-4498.2025.01.013
基于改进Swin Transformer与多模型融合的结构面几何参数解析方法
林鹏1, 2, 夏覃永1, 2, 孙鸿强1, 2, 彭鑫1, 2, 王明年1, 2, *
(1. 西南交通大学土木工程学院, 四川 成都 610031;2. 西南交通大学 交通隧道工程教育部重点实验室, 四川 成都 610031)
Structural Plane Geometric Parameter Analysis Method Based on Improved Swin Transformer and Multi-Model Fusion
LIN Peng1, 2, XIA Qinyong1, 2, SUN Hongqiang1, 2, PENG Xin1, 2, WANG Mingnian1, 2, *
(1. School of Civil Engineering, Southwest Jiaotong University, Chengdu 610031, Sichuan, China; 2. Key Laboratory of Transportation Tunnel Engineering, the Ministry of Education, Southwest Jiaotong University, Chengdu 610031, Sichuan, China)
摘要:
为解决在隧道开挖及施工过程中掌子面岩体结构面几何参数判识存在人工判读主观性强、效率低等问题,利用计算机视觉技术实现结构面判识与几何参数解析。首先,通过改进神经网络模型实现掌子面轮廓和结构面分割;然后,通过2种集成学习方法对5个结构面分割模型进行融合;最后,利用图像处理技术解析得到掌子面岩体的结构面条数、组数和平均间距等几何参数。结果表明: 1)采用U2-Net语义分割模型实现轮廓面分割任务,模型的评价指标精确率p、召回率r、f1分数分别为0.929、0.926、0.928; 2)采用5个以Swin Transformer模型为框架改进的基模型实现结构面分割,其中,SwinT-Upernet基模型的交并比(IIoU)和平均交并比(MIoU)分别达到了66.95%和81.72%,Resnet50-Upernet基模型的平均像素准确率(MAcc)达到了88.33%;3)加权平均法集成的结构面组数解析准确率达到了82.9%,结构面平均间距解析相对误差为16.15%。