- CSCD核心中文核心科技核心
- RCCSE(A+)公路运输高质量期刊T1
- Ei CompendexScopusWJCI
- EBSCOPж(AJ)JST
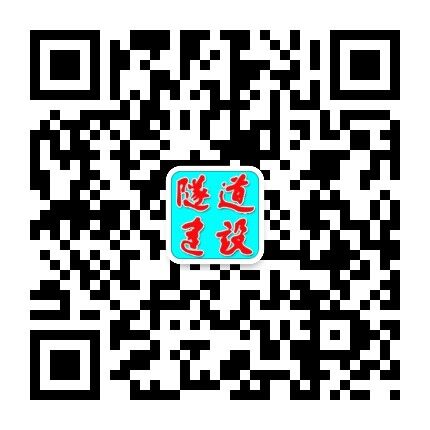
隧道建设(中英文) ›› 2014, Vol. 34 ›› Issue (4): 387-391.DOI: 10.3973/j.issn.1672-741X.2014.04.016
李宏波1,2, 周建军1,2, 王助锋1,2, 张合沛1,2, 任颖莹1,2
LI Hongbo1,2, ZHOU Jianjun1,2, WANG Zhufeng1,2, ZHANG Hepei1,2, REN Yingying1,2
摘要:
以盾构滚刀岩机作用实验台为研究对象,提出一种基于相空间重构和最小二乘支持向量机的盾构轴承状态评估及预测方法。该方法将一维时间序列重构到高维相空间中,利用相点作为支持向量机输入,自适应地对特征进行选取,并结合支持向量机非线性回归的优点,可有效预测轴承的运行状态。对实际采集的盾构滚刀岩机实验台的轴承信号进行研究分析,发现本算法的预测结果明显优于BP神经网络。将本文算法应用于工程实践,可以对盾构关键轴承状态评估和预测,能够为盾构轴承的定期保养和维修提供有效的指导。