- CSCD核心中文核心科技核心
- RCCSE(A+)公路运输高质量期刊T1
- Ei CompendexScopusWJCI
- EBSCOPж(AJ)JST
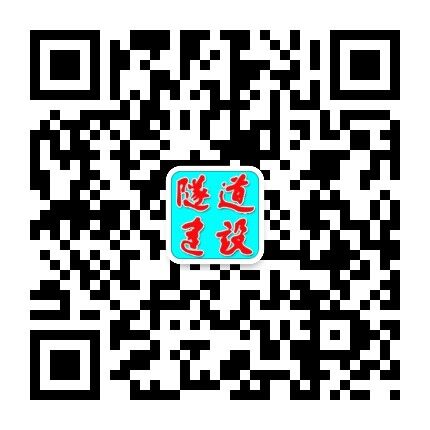
隧道建设(中英文) ›› 2019, Vol. 39 ›› Issue (7): 1132-1140.DOI: 10.3973/j.issn.2096-4498.2019.07.009
刘建东, 郭京波, 王旭东
LIU Jiandong, GUO Jingbo, WANG Xudong
摘要:
为了在盾构掘进过程中准确识别孤石地层,保证施工安全,运用理论分析与工程数据验证,研究孤石地层识别方法。基于盾构掘进比能,结合主掘进参数,提出修正比能(SM),构建孤石地层SM识别模型及识别矩阵。运用BP神经网络技术,以实测掘进参数作为训练样本,建立孤石地层神经网络识别模型,具有极高的识别精度。利用盾构掘进数据对孤石地层识别方法进行工程验证。研究结果表明: 1) 修正比能法具有较强的容错能力、稳定性及特异性,识别效果优于掘进比能法; 2) 2组实测数据下,神经网络识别结果与识别矩阵的吻合率达到98.3%和98.8%; 3) 以修正比能法为基础,结合神经网络法作为辅助与参考对孤石地层进行双重识别,具有较好的工程实际意义。
中图分类号: