- CSCD核心中文核心科技核心
- RCCSE(A+)公路运输高质量期刊T1
- Ei CompendexScopusWJCI
- EBSCOPж(AJ)JST
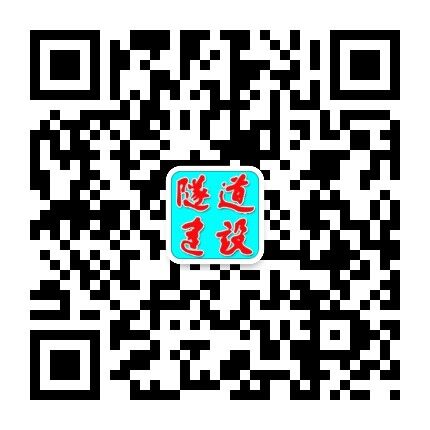
隧道建设(中英文) ›› 2021, Vol. 41 ›› Issue (2): 199-205.DOI: 10.3973/j.issn.2096-4498.2021.02.005
李增良
LI Zengliang
摘要: 在盾构施工过程中准确预测施工引起的地表变形,对于保障盾构施工的顺利掘进具有重要意义。基于此,提出盾构施工地表变形MIC-LSTM动态预测模型。首先,确定影响地表变形的主要因素,并采用最大信息系数法(MIC,maximal information coefficient)确定各个影响因素和地表变形之间的相关程度,进而对各个影响因素赋权; 其次,将赋权后的各个影响因素和盾构中心处过去最近10个监测时刻的地表变形数据作为输入变量、未来3个监测时刻的变形数据作为输出变量来构建长短期记忆(LSTM,long shortterm memory)神经网络动态预测模型;最后,为验证所构建的MIC-LSTM动态预测模型的实用性,依托昆明地铁5号线盾构施工项目,将预测结果与LSTM、RNN(recurrent neural network)以及BP(back propagation)神经网络的预测结果进行对比。研究结果表明: 所构建的盾构施工地表变形动态预测模型具有较高的预测精度。
中图分类号: