- 中国科学引文数据库(CSCD)来源期刊
- 中文核心期刊中文科技核心期刊
- Scopus RCCSE中国核心学术期刊
- 美国EBSCO数据库 俄罗斯《文摘杂志》
- 《日本科学技术振兴机构数据库(中国)》
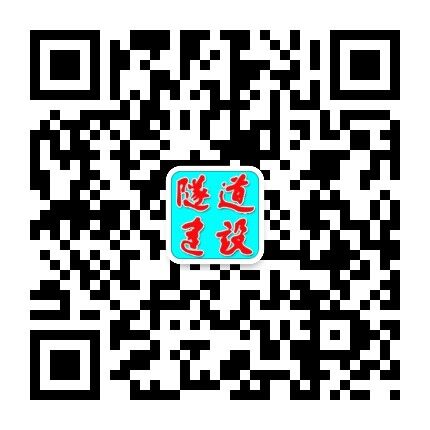
隧道建设(中英文) ›› 2023, Vol. 43 ›› Issue (3): 408-416.DOI: 10.3973/j.issn.2096-4498.2023.03.005
刘四进1, 王宇博2, 方勇2, *, 熊英健2, 马浴阳1
(1. 中铁十四局集团有限公司, 山东 济南 250101;2. 西南交通大学 交通隧道工程教育部重点实验室, 四川 成都 610031)
Prediction Model for Grease Consumption of Super-Large Diameter Slurry Shield Based on Bidirectional Long Short-Term Memory and Autoregressive Integrated Moving Average Model
LIU Sijin1, WANG Yubo2, FANG Yong2, *, XIONG Yingjian2, MA Yuyang1
(1.China Railway 14th Bureau Group Co., Ltd., Jinan 250101, Shandong, China; 2. Key Laboratory of Transportation Tunnel Engineering, Ministry of Education, Southwest Jiaotong University, Chengdu 610031, Sichuan, China)
摘要: 为了对盾构盾尾油脂消耗控制提供指导,以盾尾密封油脂消耗量预测精度为目标,采用双向LSTM与ARIMA模型相结合的方法,构建Bi-LSTM-ARIMA盾尾密封油脂消耗时间序列预测模型,在综合考虑盾构掘进参数与相关工程地质及水文地质参数的基础上,建立盾尾密封油脂消耗BP神经网络预测模型。以济南黄河隧道为依托,基于区间隧道既有盾尾油脂消耗数据对盾构东线区间盾尾密封油脂消耗量进行训练和预测。研究结果表明: Bi-LSTM-ARIMA模型对盾尾密封油脂消耗预测的均方根误差为13.47,平均相对误差仅为3.13%,相较于ARIMA时间序列模型和BP神经网络模型具有更高的预测精度,具有较好的实用性与可靠性。