- 中国科学引文数据库(CSCD)来源期刊
- 中文核心期刊中文科技核心期刊
- Scopus RCCSE中国核心学术期刊
- 美国EBSCO数据库 俄罗斯《文摘杂志》
- 《日本科学技术振兴机构数据库(中国)》
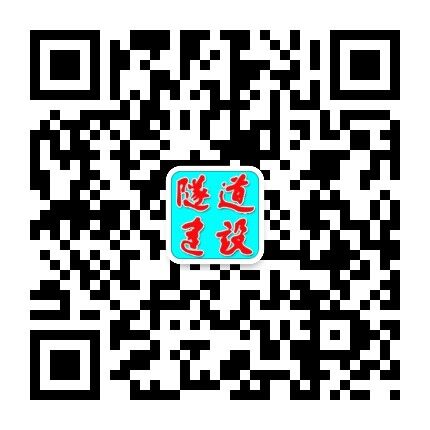
隧道建设(中英文) ›› 2021, Vol. 41 ›› Issue (S2): 246-254.DOI: 10.3973/j.issn.2096-4498.2021.S2.031
刘春梅1, 姜巍1, 宫亚峰2, *, 林思远2
LIU Chunmei1, JIANG Wei1, GONG Yafeng2, *, LIN Siyuan2
(1. China Railway Tunnel Group Road & Bridge Engineering Co., Ltd., Tianjin 300308, China; 2. Transportation College of Jilin University, Changchun 130022, Jilin, China)
摘要: 为研究深基坑开挖过程中的受力变形规律,以长春地铁34 m深基坑为研究对象,采用MIDAS GTS对各个工况下基坑开挖进行模拟研究,得到不同工况下地表沉降、围护结构变形、轴力变化规律,将模拟数据与实际监测数据进行对比,验证模型的准确性,并根据所建立的模型提取各工况下最大地表沉降值,以开挖深度、支撑数量和降水量作为输入参数,对深基坑地表沉降进行预测。首先将模型提取的地表沉降值作为样本数据,通过K均值聚类算法将样本数据进行归类,为径向基神经网络确定基函数中心,构建K均值聚类算法K-RBF预测模型,进而对地表沉降进行预测。根据工程实例结果表明,K-RBF预测模型较普通RBF模型更接近实际监测数据,与实际监测数据相比,K-RBF的均方误差为0.212 mm、平均绝对误差为0.278 mm、最大绝对误差为1.11 mm,具有较好的预测精度。
中图分类号: