- CSCD核心中文核心科技核心
- RCCSE(A+)公路运输高质量期刊T1
- Ei CompendexScopusWJCI
- EBSCOPж(AJ)JST
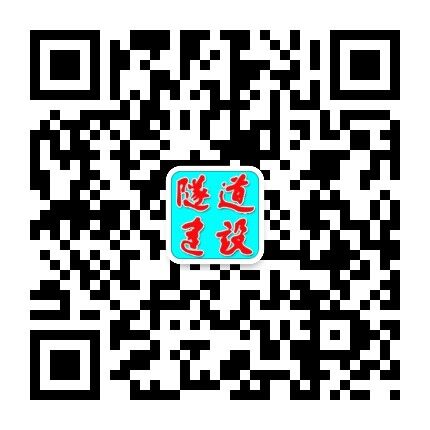
隧道建设(中英文) ›› 2024, Vol. 44 ›› Issue (S2): 286-299.DOI: 10.3973/j.issn.2096-4498.2024.S2.028
于维鹤1, 黄志强2, *, 王乃毅2, 张宁2
(1. 中国石油辽宁丹东销售分公司, 辽宁 丹东 118016; 2. 沈阳工业大学建筑与土木工程学院, 辽宁 沈阳 110870)
Application of Improved Pelican Optimization Algorithm-Convolutional Neural Networks-Bidirectional Gated Recurrent Unit Model in Predicting Foundation Pit Dewatering
YU Weihe1, HUANG Zhiqiang2, *, WANG Naiyi2, ZHANG Ning2
(1. PetroChina Liaoning Dandong Sales Branch, Dandong 118016, Liaoning, China; 2. School of Architecture and Civil Engineering, Shenyang University of Technology, Shenyang 110870, Liaoning, China)
摘要: 为提高基坑降水水位预测精度,加强对基坑降水水位的控制,在标准鹈鹕优化算法(POA)的基础上引入Logistic混沌映射、自适应动态权重因子w及萤火虫扰动最优位置3种优化策略,提出改进鹈鹕优化算法(IPOA)。选取4种基准测试函数对IPOA算法进行仿真测试,并选用其他5种优化算法进行对比分析。以沈阳北站基坑降水工程为实例进行研究,采用IPOA算法优化CNN-BiGRU模型中的隐含层节点个数、初始学习率以及L2正则化系数,形成IPOA-CNN-BiGRU水位预测模型,选用GWO、WOA、POA算法优化CNN-BiGRU模型并搭建Midas GTS NX基坑降水有限元数值模拟模型,进行对比分析,验证本文提出的预测模型在水位预测任务中的优越性。结果表明: 1)IPOA优化算法在单峰及多峰函数的寻优任务中均体现出较好的性能,其在4种基准测试函数中的寻优速度更快,寻优结果要明显优于其余5种算法。2)在水位预测任务中,IPOA-CNN-BiGRU水位预测模型的EMAE、ERMSE、EMAPE分别为0.213 8、0.253 9、0.771 3,预测精度比GWO、WOA、POA优化下的CNN-BiGRU模型分别提高了32.36%、20.96%、15.79%。3)相比Midas GTS NX有限元模型,IPOA-CNN-BiGRU模型的参数依赖性更低,其预测精度提高了80.65%,验证了采用IPOA-CNN-BiGRU模型对基坑降水水位进行预测的可行性。